All Common Core: High School - Statistics and Probability Resources
Example Questions
Example Question #1 : Plotting And Analyzing Residuals: Ccss.Math.Content.Hss Id.B.6b
A researcher for a motor vehicle company wants to observe the relationship between a vehicle's weight and mileage. He decides to investigate 40 vehicles and tabulates the following data.
Afterwards, he plotted the data into a scatter plot and fitted a trendline to the graph.
Which of the following is the best conclusion that can be made about the data's linearity?
The graph is not linear because the plot of the residuals possesses a U-shaped distribution.
The graph is linear because the plot of the residuals possesses a U-shaped distribution.
The graph is not linear because the plot of the residuals possesses a random distribution.
None of these
The graph is linear because the plot of the residuals possesses a random distribution.
The graph is linear because the plot of the residuals possesses a random distribution.
When points are plotted in a linear regression model, trendlines or best-fit lines are used to make inferences and predictions about the data. There are several common trendline types: logarithmic, polynomial, exponential, power, and linear. Contrary to popular belief, a linear trendline is not always the best fit for every data set. In other words, we need to test the trendline to figure out whether or not it possesses strong associations of linearity between points. We can test this by graphing the plot’s residuals.
What is meant by “residuals”? The residual of a point on a graph is calculated by subtracting the predicted y-value from its actual value. It is written using the following equation:
In this equation:
The actual values are represented by the points plotted on the graph, while the predicted values are represented by the trend line. The difference between each actual value and its predicted counterpart is the point's residual.
The question provided a table of the x- and y-values for the scatterplot. It also provided the equation of the linear trendline. Given this information, we can calculate the predicted y-values and the residuals of the scatterplot.
Let’s start by calculating the predicted y-values using the equation of the trendline and the x-values.
Lets start with the first x-value:
Now, calculate each predicted value for every x-coordinate in the scatter plot. Afterwards, calculate the residual for each point. For example,
Calculate the residuals for every point in the graph.
Now, we have calculated the predicted y-values and the residuals; therefore, we can create a graph of the residuals in the series. The graph will contain the residual values on the y-axis and the original x-values on the x-axis.
Now, we can fit a trendline to the data. Notice that in this case the trendline is nearly horizontal. This indicates that there is a random spread in the residual data, which indicates that there is a linear correlation between points. The correct answer is "The graph is linear because the plot of the residuals possesses a random distribution." Now, we can determine a scatter plot's linearity using a graph of the plot's residuals.
Example Question #1 : Plotting And Analyzing Residuals: Ccss.Math.Content.Hss Id.B.6b
A researcher for a motor vehicle company wants to observe the relationship between a vehicle's weight and mileage. He decides to investigate 40 vehicles and tabulates the following data.
Which of the following is the best conclusion that can be made about the data's linearity?
The graph is linear because the plot of the residuals possesses a U-shaped distribution.
The graph is linear because the plot of the residuals possesses a random distribution.
The graph is not linear because the plot of the residuals possesses a U-shaped distribution.
None of these
The graph is not linear because the plot of the residuals possesses a random distribution.
The graph is not linear because the plot of the residuals possesses a U-shaped distribution.
When points are plotted in a linear regression model, trendlines or best-fit lines are used to make inferences and predictions about the data. There are several common trendline types: logarithmic, polynomial, exponential, power, and linear. Contrary to popular belief, a linear trendline is not always the best fit for every data set. In other words, we need to test the trendline to figure out whether or not it possesses strong associations of linearity between points. We can test this by graphing the plot’s residuals.
What is meant by “residuals”? The residual of a point on a graph is calculated by subtracting the predicted y-value from its actual value. It is written using the following equation:
In this equation
The actual values are represented by the points plotted on the graph, while the predicted values are represented by the trend line. The difference between each actual value and its predicted counterpart is the point's residual.
The question provided a table of the x- and y-values for the scatterplot. It also provided the equation of the linear trendline. Given this information, we can calculate the predicted y-values and the residuals of the scatterplot.
Let’s start by calculating the predicted y-values using the equation of the trendline and the x-values.
Lets start with the first x-value:
Now, calculate each predicted value for every x-coordinate in the scatter plot. Afterwards, calculate the residual for each point. For example,
Calculate the residuals for every point in the graph.
FIRST 20 items in Table
Now, we have calculated the predicted y-values and the residuals; therefore, we can create a graph of the residuals in the series. The graph will contain the residual values on the y-axis and the original x-values on the x-axis.
Let's observe the data. We can see that when the residual data is plotted on the graph, it forms a U-shaped distribution. If the plot of the residuals form a U-shaped distribution, then the graph is not linear. The correct answer is 'The graph is not linear because the plot of the residuals possesses a U-shaped distribution.' Now, we can determine a scatter plot's linearity using a graph of the plot's residuals.
Example Question #1 : Plotting And Analyzing Residuals: Ccss.Math.Content.Hss Id.B.6b
A researcher for a motor vehicle company wants to observe the relationship between a vehicle's weight and mileage. He decides to investigate 40 vehicles and tabulates the following data.
Which of the following is the best conclusion that can be made about the data's linearity?
The graph is linear because the plot of the residuals possesses a random distribution.
None of these
The graph is linear because the plot of the residuals possesses a U-shaped distribution.
The graph is not linear because the plot of the residuals possesses a random distribution.
The graph is not linear because the plot of the residuals possesses a U-shaped distribution.
The graph is not linear because the plot of the residuals possesses a U-shaped distribution.
When points are plotted in a linear regression model, trendlines or best-fit lines are used to make inferences and predictions about the data. There are several common trendline types: logarithmic, polynomial, exponential, power, and linear. Contrary to popular belief, a linear trendline is not always the best fit for every data set. In other words, we need to test the trendline to figure out whether or not it possesses strong associations of linearity between points. We can test this by graphing the plot’s residuals.
What is meant by “residuals”? The residual of a point on a graph is calculated by subtracting the predicted y-value from its actual value. It is written using the following equation:
In this equation
The actual values are represented by the points plotted on the graph, while the predicted values are represented by the trend line. The difference between each actual value and its predicted counterpart is the point's residual.
The question provided a table of the x- and y-values for the scatterplot. It also provided the equation of the linear trendline. Given this information, we can calculate the predicted y-values and the residuals of the scatterplot.
Let’s start by calculating the predicted y-values using the equation of the trendline and the x-values.
Lets start with the first x-value:
Now, calculate each predicted value for every x-coordinate in the scatter plot. Afterwards, calculate the residual for each point. For example,
Calculate the residuals for every point in the graph.
Now, we have calculated the predicted y-values and the residuals; therefore, we can create a graph of the residuals in the series. The graph will contain the residual values on the y-axis and the original x-values on the x-axis.
Let's observe the data. We can see that when the residual data is plotted on the graph, it forms a U-shaped distribution. If the plot of the residuals form a U-shaped distribution, then the graph is not linear. The correct answer is 'The graph is not linear because the plot of the residuals possesses a U-shaped distribution.' Now, we can determine a scatter plot's linearity using a graph of the plot's residuals.
Example Question #5 : Plotting And Analyzing Residuals: Ccss.Math.Content.Hss Id.B.6b
A researcher for a motor vehicle company wants to observe the relationship between a vehicle's weight and mileage. He decides to investigate 40 vehicles and tabulates the following data.
Which of the following is the best conclusion that can be made about the data's linearity?
None of these
The graph is not linear because the plot of the residuals possesses a U-shaped distribution.
The graph is not linear because the plot of the residuals possesses a random distribution.
The graph is linear because the plot of the residuals possesses a U-shaped distribution.
The graph is linear because the plot of the residuals possesses a random distribution.
The graph is not linear because the plot of the residuals possesses a U-shaped distribution.
When points are plotted in a linear regression model, trendlines or best-fit lines are used to make inferences and predictions about the data. There are several common trendline types: logarithmic, polynomial, exponential, power, and linear. Contrary to popular belief, a linear trendline is not always the best fit for every data set. In other words, we need to test the trendline to figure out whether or not it possesses strong associations of linearity between points. We can test this by graphing the plot’s residuals.
What is meant by “residuals”? The residual of a point on a graph is calculated by subtracting the predicted y-value from its actual value. It is written using the following equation:
In this equation
The actual values are represented by the points plotted on the graph, while the predicted values are represented by the trend line. The difference between each actual value and its predicted counterpart is the point's residual.
The question provided a table of the x- and y-values for the scatterplot. It also provided the equation of the linear trendline. Given this information, we can calculate the predicted y-values and the residuals of the scatterplot.
Let’s start by calculating the predicted y-values using the equation of the trendline and the x-values.
Lets start with the first x-value:
Now, calculate each predicted value for every x-coordinate in the scatter plot. Afterwards, calculate the residual for each point. For example,
Calculate the residuals for every point in the graph.
Now, we have calculated the predicted y-values and the residuals; therefore, we can create a graph of the residuals in the series. The graph will contain the residual values on the y-axis and the original x-values on the x-axis.
Let's observe the data. We can see that when the residual data is plotted on the graph, it forms a U-shaped distribution. If the plot of the residuals form a U-shaped distribution, then the graph is not linear. The correct answer is 'The graph is not linear because the plot of the residuals possesses a U-shaped distribution.' Now, we can determine a scatter plot's linearity using a graph of the plot's residuals.
Example Question #6 : Plotting And Analyzing Residuals: Ccss.Math.Content.Hss Id.B.6b
A researcher for a motor vehicle company wants to observe the relationship between a vehicle's weight and mileage. He decides to investigate 40 vehicles and tabulates the following data.
Which of the following is the best conclusion that can be made about the data's linearity?
The graph is not linear because the plot of the residuals possesses a random distribution.
The graph is linear because the plot of the residuals possesses a random distribution.
The graph is linear because the plot of the residuals possesses a U-shaped distribution.
The graph is not linear because the plot of the residuals possesses a U-shaped distribution.
None of these
The graph is linear because the plot of the residuals possesses a random distribution.
When points are plotted in a linear regression model, trendlines or best-fit lines are used to make inferences and predictions about the data. There are several common trendline types: logarithmic, polynomial, exponential, power, and linear. Contrary to popular belief, a linear trendline is not always the best fit for every data set. In other words, we need to test the trendline to figure out whether or not it possesses strong associations of linearity between points. We can test this by graphing the plot’s residuals.
What is meant by “residuals”? The residual of a point on a graph is calculated by subtracting the predicted y-value from its actual value. It is written using the following equation:
In this equation
The actual values are represented by the points plotted on the graph, while the predicted values are represented by the trend line. The difference between each actual value and its predicted counterpart is the point's residual.
The question provided a table of the x- and y-values for the scatterplot. It also provided the equation of the linear trendline. Given this information, we can calculate the predicted y-values and the residuals of the scatterplot.
Let’s start by calculating the predicted y-values using the equation of the trendline and the x-values.
Lets start with the first x-value:
Now, calculate each predicted value for every x-coordinate in the scatter plot. Afterwards, calculate the residual for each point. For example,
Calculate the residuals for every point in the graph.
Now, we have calculated the predicted y-values and the residuals; therefore, we can create a graph of the residuals in the series. The graph will contain the residual values on the y-axis and the original x-values on the x-axis.
Now, we can fit a trendline to the data. Notice that in this case the trendline is nearly horizontal. This indicates that there is a random spread in the residual data, which indicates that there is a linear correlation between points. The correct answer is "The graph is linear because the plot of the residuals possesses a random distribution." Now, we can determine a scatter plot's linearity using a graph of the plot's residuals.
Example Question #7 : Plotting And Analyzing Residuals: Ccss.Math.Content.Hss Id.B.6b
A researcher for a motor vehicle company wants to observe the relationship between a vehicle's weight and mileage. He decides to investigate 40 vehicles and tabulates the following data.
Which of the following is the best conclusion that can be made about the data's linearity?
None of these
The graph is not linear because the plot of the residuals possesses a U-shaped distribution.
The graph is linear because the plot of the residuals possesses a random distribution.
The graph is linear because the plot of the residuals possesses a U-shaped distribution.
The graph is not linear because the plot of the residuals possesses a random distribution.
The graph is linear because the plot of the residuals possesses a random distribution.
When points are plotted in a linear regression model, trendlines or best-fit lines are used to make inferences and predictions about the data. There are several common trendline types: logarithmic, polynomial, exponential, power, and linear. Contrary to popular belief, a linear trendline is not always the best fit for every data set. In other words, we need to test the trendline to figure out whether or not it possesses strong associations of linearity between points. We can test this by graphing the plot’s residuals.
What is meant by “residuals”? The residual of a point on a graph is calculated by subtracting the predicted y-value from its actual value. It is written using the following equation:
In this equation
The actual values are represented by the points plotted on the graph, while the predicted values are represented by the trend line. The difference between each actual value and its predicted counterpart is the point's residual.
The question provided a table of the x- and y-values for the scatterplot. It also provided the equation of the linear trendline. Given this information, we can calculate the predicted y-values and the residuals of the scatterplot.
Let’s start by calculating the predicted y-values using the equation of the trendline and the x-values.
Lets start with the first x-value:
Now, calculate each predicted value for every x-coordinate in the scatter plot. Afterwards, calculate the residual for each point. For example,
Calculate the residuals for every point in the graph.
Now, we have calculated the predicted y-values and the residuals; therefore, we can create a graph of the residuals in the series. The graph will contain the residual values on the y-axis and the original x-values on the x-axis.
Now, we can fit a trendline to the data. Notice that in this case the trendline is nearly horizontal. This indicates that there is a random spread in the residual data, which indicates that there is a linear correlation between points. The correct answer is "The graph is linear because the plot of the residuals possesses a random distribution." Now, we can determine a scatter plot's linearity using a graph of the plot's residuals.
Example Question #8 : Plotting And Analyzing Residuals: Ccss.Math.Content.Hss Id.B.6b
A researcher for a motor vehicle company wants to observe the relationship between a vehicle's weight and mileage. He decides to investigate 40 vehicles and tabulates the following data.
Which of the following is the best conclusion that can be made about the data's linearity?
None of these
The graph is not linear because the plot of the residuals possesses a U-shaped distribution.
The graph is linear because the plot of the residuals possesses a random distribution.
The graph is linear because the plot of the residuals possesses a U-shaped distribution.
The graph is not linear because the plot of the residuals possesses a random distribution.
The graph is linear because the plot of the residuals possesses a random distribution.
When points are plotted in a linear regression model, trendlines or best-fit lines are used to make inferences and predictions about the data. There are several common trendline types: logarithmic, polynomial, exponential, power, and linear. Contrary to popular belief, a linear trendline is not always the best fit for every data set. In other words, we need to test the trendline to figure out whether or not it possesses strong associations of linearity between points. We can test this by graphing the plot’s residuals.
What is meant by “residuals”? The residual of a point on a graph is calculated by subtracting the predicted y-value from its actual value. It is written using the following equation:
In this equation
The actual values are represented by the points plotted on the graph, while the predicted values are represented by the trend line. The difference between each actual value and its predicted counterpart is the point's residual.
The question provided a table of the x- and y-values for the scatterplot. It also provided the equation of the linear trendline. Given this information, we can calculate the predicted y-values and the residuals of the scatterplot.
Let’s start by calculating the predicted y-values using the equation of the trendline and the x-values.
Lets start with the first x-value:
Now, calculate each predicted value for every x-coordinate in the scatter plot. Afterwards, calculate the residual for each point. For example,
Calculate the residuals for every point in the graph.
Now, we have calculated the predicted y-values and the residuals; therefore, we can create a graph of the residuals in the series. The graph will contain the residual values on the y-axis and the original x-values on the x-axis.
Now, we can fit a trendline to the data. Notice that in this case the trendline is nearly horizontal. This indicates that there is a random spread in the residual data, which indicates that there is a linear correlation between points. The correct answer is "The graph is linear because the plot of the residuals possesses a random distribution." Now, we can determine a scatter plot's linearity using a graph of the plot's residuals.
Example Question #9 : Plotting And Analyzing Residuals: Ccss.Math.Content.Hss Id.B.6b
A researcher for a motor vehicle company wants to observe the relationship between a vehicle's weight and mileage. He decides to investigate 40 vehicles and tabulates the following data.
Which of the following is the best conclusion that can be made about the data's linearity?
The graph is linear because the plot of the residuals possesses a random distribution.
None of these
The graph is linear because the plot of the residuals possesses a U-shaped distribution.
The graph is not linear because the plot of the residuals possesses a U-shaped distribution.
The graph is not linear because the plot of the residuals possesses a random distribution.
The graph is not linear because the plot of the residuals possesses a U-shaped distribution.
When points are plotted in a linear regression model, trendlines or best-fit lines are used to make inferences and predictions about the data. There are several common trendline types: logarithmic, polynomial, exponential, power, and linear. Contrary to popular belief, a linear trendline is not always the best fit for every data set. In other words, we need to test the trendline to figure out whether or not it possesses strong associations of linearity between points. We can test this by graphing the plot’s residuals.
What is meant by “residuals”? The residual of a point on a graph is calculated by subtracting the predicted y-value from its actual value. It is written using the following equation:
In this equation
The actual values are represented by the points plotted on the graph, while the predicted values are represented by the trend line. The difference between each actual value and its predicted counterpart is the point's residual.
The question provided a table of the x- and y-values for the scatterplot. It also provided the equation of the linear trendline. Given this information, we can calculate the predicted y-values and the residuals of the scatterplot.
Let’s start by calculating the predicted y-values using the equation of the trendline and the x-values.
Lets start with the first x-value:
Now, calculate each predicted value for every x-coordinate in the scatter plot. Afterwards, calculate the residual for each point. For example,
Calculate the residuals for every point in the graph.
Now, we have calculated the predicted y-values and the residuals; therefore, we can create a graph of the residuals in the series. The graph will contain the residual values on the y-axis and the original x-values on the x-axis.
Let's observe the data. We can see that when the residual data is plotted on the graph, it forms a U-shaped distribution. If the plot of the residuals form a U-shaped distribution, then the graph is not linear. The correct answer is 'The graph is not linear because the plot of the residuals possesses a U-shaped distribution.' Now, we can determine a scatter plot's linearity using a graph of the plot's residuals.
Example Question #10 : Plotting And Analyzing Residuals: Ccss.Math.Content.Hss Id.B.6b
A researcher for a motor vehicle company wants to observe the relationship between a vehicle's weight and mileage. He decides to investigate 40 vehicles and tabulates the following data.
Which of the following is the best conclusion that can be made about the data's linearity?
The graph is not linear because the plot of the residuals possesses a random distribution.
The graph is linear because the plot of the residuals possesses a U-shaped distribution.
The graph is linear because the plot of the residuals possesses a random distribution.
None of these
The graph is not linear because the plot of the residuals possesses a U-shaped distribution.
The graph is not linear because the plot of the residuals possesses a U-shaped distribution.
When points are plotted in a linear regression model, trendlines or best-fit lines are used to make inferences and predictions about the data. There are several common trendline types: logarithmic, polynomial, exponential, power, and linear. Contrary to popular belief, a linear trendline is not always the best fit for every data set. In other words, we need to test the trendline to figure out whether or not it possesses strong associations of linearity between points. We can test this by graphing the plot’s residuals.
What is meant by “residuals”? The residual of a point on a graph is calculated by subtracting the predicted y-value from its actual value. It is written using the following equation:
In this equation
The actual values are represented by the points plotted on the graph, while the predicted values are represented by the trend line. The difference between each actual value and its predicted counterpart is the point's residual.
The question provided a table of the x- and y-values for the scatterplot. It also provided the equation of the linear trendline. Given this information, we can calculate the predicted y-values and the residuals of the scatterplot.
Let’s start by calculating the predicted y-values using the equation of the trendline and the x-values.
Lets start with the first x-value:
Now, calculate each predicted value for every x-coordinate in the scatter plot. Afterwards, calculate the residual for each point. For example,
\\ \\ e=y-\^y
\\ e=52-39.0202 \\ e=88.3212
Calculate the residuals for every point in the graph.
Now, we have calculated the predicted y-values and the residuals; therefore, we can create a graph of the residuals in the series. The graph will contain the residual values on the y-axis and the original x-values on the x-axis.
Let's observe the data. We can see that when the residual data is plotted on the graph, it forms a U-shaped distribution. If the plot of the residuals form a U-shaped distribution, then the graph is not linear. The correct answer is 'The graph is not linear because the plot of the residuals possesses a U-shaped distribution.' Now, we can determine a scatter plot's linearity using a graph of the plot's residuals.
Example Question #121 : Interpreting Categorical & Quantitative Data
A researcher for a motor vehicle company wants to observe the relationship between a vehicle's weight and mileage. He decides to investigate 40 vehicles and tabulates the following data.
Which of the following is the best conclusion that can be made about the data's linearity?
The graph is linear because the plot of the residuals possesses a random distribution.
The graph is linear because the plot of the residuals possesses a U-shaped distribution.
The graph is not linear because the plot of the residuals possesses a U-shaped distribution.
The graph is not linear because the plot of the residuals possesses a random distribution.
None of these
The graph is linear because the plot of the residuals possesses a random distribution.
When points are plotted in a linear regression model, trendlines or best-fit lines are used to make inferences and predictions about the data. There are several common trendline types: logarithmic, polynomial, exponential, power, and linear. Contrary to popular belief, a linear trendline is not always the best fit for every data set. In other words, we need to test the trendline to figure out whether or not it possesses strong associations of linearity between points. We can test this by graphing the plot’s residuals.
What is meant by “residuals”? The residual of a point on a graph is calculated by subtracting the predicted y-value from its actual value. It is written using the following equation:
In this equation
The actual values are represented by the points plotted on the graph, while the predicted values are represented by the trend line. The difference between each actual value and its predicted counterpart is the point's residual.
The question provided a table of the x- and y-values for the scatterplot. It also provided the equation of the linear trendline. Given this information, we can calculate the predicted y-values and the residuals of the scatterplot.
Let’s start by calculating the predicted y-values using the equation of the trendline and the x-values.
Lets start with the first x-value:
Now, calculate each predicted value for every x-coordinate in the scatter plot. Afterwards, calculate the residual for each point. For example,
Calculate the residuals for every point in the graph.
Now, we have calculated the predicted y-values and the residuals; therefore, we cancreate a graph of the residuals in the series. The graph will contain the residual values on the y-axis and the original x-values on the x-axis.
Now, we can fit a trendline to the data. Notice that in this case the trendline is nearly horizontal. This indicates that there is a random spread in the residual data, which indicates that there is a linear correlation between points. The correct answer is "The graph is linear because the plot of the residuals possesses a random distribution." Now, we can determine a scatter plot's linearity using a graph of the plot's residuals.
Certified Tutor
All Common Core: High School - Statistics and Probability Resources
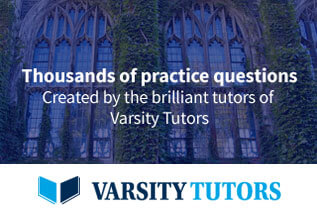