All Common Core: High School - Statistics and Probability Resources
Example Questions
Example Question #7 : General Multiplication Rule In A Uniform Probability Model: Ccss.Math.Content.Hss Cp.B.8
Use the general multiplication rule to solve for the given probability model. For events A and B, calculate P(A\cap B) given the following information:
Cannot be determined
In order to solve this problem, we need to discuss probabilities, conditional probabilities, and the general multiplication rule of probability. A probability is generally defined as the chances or likelihood of an event occurring. It is calculated by identifying two components: the event and the sample space. The event is defined as the favorable outcome or success that we wish to observe. On the other hand, the sample space is defined as the set of all possible outcomes for the event. Mathematically we calculate probabilities by dividing the event by the sample space:
Let's use a simple example: the rolling of a die. We want to know the probability of rolling a one. We know that the sample space is six because there are six sides or outcomes to the die. Also, we know that there is only a single side with a value of one; therefore,
Now, let's convert this into a percentage:
Probabilities expressed in fraction form will have values between zero and one. One indicates that an event will definitely occur, while zero indicates that an event will not occur. Likewise, probabilities expressed as percentages possess values between zero and one hundred percent where probabilities closer to zero are unlikely to occur and those close to one hundred percent are more likely to occur.
Now that we understand the definition of a probability in its most general sense, we can investigate conditional probabilities. A conditional probability is defined as the probability that event B will occur given the information that an event A has already occurred.
It is expressed using the following equation:
In this equation, the probability of event B given that event A has occurred is equal to the probability of the intersection of events A and B divided by the probability of event A. It is important to note that if the events are independent, then the probability of event B given event A is simply the probability of event B because event A does not affect it.
Before we can use this information to discuss the general multiplication rule of probability, we must understand what is meant by the terms uniform and non-uniform in a probability model. In a uniform probability mode all events possess an equal chance of occurring. For instance, rolling any one side on a six sided fair die will have the same probability: one out of six. Likewise, the probability of a spinner landing on any of the colored spaces in the uniform figure is ten percent; therefore, uniform probability models posses events that all have the same probability of occurring.
On the other hand, in non-uniform models events do not share an equal probabilities. For instance, someone may weight or alter a die in order to increase the chances of rolling a particular number. Likewise, the probability of a spinner landing on each of the colored spaces in the non-uniform image possess differing probabilities; thus, the probabilities of non-uniform events differ from one another.
Now that we understand what is meant by uniform and non-uniform probability models, we can discuss the general multiplication role of probability. First, it can only be used to answer questions regarding uniform models because non-uniform models require more advanced mathematics. Second, the formula is based upon the conditional probability formula. Let's start by reviewing this formula.
Let's begin by multiplying each side of the equation by the following probability:
Cross out like terms and simplify in order to create our first rule of general multiplication of probabilities.
Next, we can use the same model to create another multiplication formula for the conditional probability:
Simplify as before in order to create our second rule of general multiplication of probabilities.
We can create a third rule of general multiplication of probabilities by setting the two equations equal to one another by using the like term:
These formulas enable us to easily solve probabilities problems that may be lacking information. Let's use this information to solve the problem. We need to find the probability of given the following information:
We will start by choosing the correct formula. In this case, we shall use the first formula:
Now, we can substitute in known values.
Solve.
Example Question #8 : General Multiplication Rule In A Uniform Probability Model: Ccss.Math.Content.Hss Cp.B.8
Use the general multiplication rule to solve for the given probability model. For events A and B, calculate P(A\cap B) given the following information:
Cannot be determined
In order to solve this problem, we need to discuss probabilities, conditional probabilities, and the general multiplication rule of probability. A probability is generally defined as the chances or likelihood of an event occurring. It is calculated by identifying two components: the event and the sample space. The event is defined as the favorable outcome or success that we wish to observe. On the other hand, the sample space is defined as the set of all possible outcomes for the event. Mathematically we calculate probabilities by dividing the event by the sample space:
Let's use a simple example: the rolling of a die. We want to know the probability of rolling a one. We know that the sample space is six because there are six sides or outcomes to the die. Also, we know that there is only a single side with a value of one; therefore,
Now, let's convert this into a percentage:
Probabilities expressed in fraction form will have values between zero and one. One indicates that an event will definitely occur, while zero indicates that an event will not occur. Likewise, probabilities expressed as percentages possess values between zero and one hundred percent where probabilities closer to zero are unlikely to occur and those close to one hundred percent are more likely to occur.
Now that we understand the definition of a probability in its most general sense, we can investigate conditional probabilities. A conditional probability is defined as the probability that event B will occur given the information that an event A has already occurred.
It is expressed using the following equation:
In this equation, the probability of event B given that event A has occurred is equal to the probability of the intersection of events A and B divided by the probability of event A. It is important to note that if the events are independent, then the probability of event B given event A is simply the probability of event B because event A does not affect it.
Before we can use this information to discuss the general multiplication rule of probability, we must understand what is meant by the terms uniform and non-uniform in a probability model. In a uniform probability mode all events possess an equal chance of occurring. For instance, rolling any one side on a six sided fair die will have the same probability: one out of six. Likewise, the probability of a spinner landing on any of the colored spaces in the uniform figure is ten percent; therefore, uniform probability models posses events that all have the same probability of occurring.
On the other hand, in non-uniform models events do not share an equal probabilities. For instance, someone may weight or alter a die in order to increase the chances of rolling a particular number. Likewise, the probability of a spinner landing on each of the colored spaces in the non-uniform image possess differing probabilities; thus, the probabilities of non-uniform events differ from one another.
Now that we understand what is meant by uniform and non-uniform probability models, we can discuss the general multiplication role of probability. First, it can only be used to answer questions regarding uniform models because non-uniform models require more advanced mathematics. Second, the formula is based upon the conditional probability formula. Let's start by reviewing this formula.
Let's begin by multiplying each side of the equation by the following probability:
Cross out like terms and simplify in order to create our first rule of general multiplication of probabilities.
Next, we can use the same model to create another multiplication formula for the conditional probability:
Simplify as before in order to create our second rule of general multiplication of probabilities.
We can create a third rule of general multiplication of probabilities by setting the two equations equal to one another by using the like term:
These formulas enable us to easily solve probabilities problems that may be lacking information. Let's use this information to solve the problem. We need to find the probability of given the following information:
We will start by choosing the correct formula. In this case, we shall use the first formula:
Now, we can substitute in known values.
Solve.
Example Question #9 : General Multiplication Rule In A Uniform Probability Model: Ccss.Math.Content.Hss Cp.B.8
Use the general multiplication rule to solve for the given probability model. For events A and B, calculate P(A\cap B) given the following information:
Cannot be determined
In order to solve this problem, we need to discuss probabilities, conditional probabilities, and the general multiplication rule of probability. A probability is generally defined as the chances or likelihood of an event occurring. It is calculated by identifying two components: the event and the sample space. The event is defined as the favorable outcome or success that we wish to observe. On the other hand, the sample space is defined as the set of all possible outcomes for the event. Mathematically we calculate probabilities by dividing the event by the sample space:
Let's use a simple example: the rolling of a die. We want to know the probability of rolling a one. We know that the sample space is six because there are six sides or outcomes to the die. Also, we know that there is only a single side with a value of one; therefore,
Now, let's convert this into a percentage:
Probabilities expressed in fraction form will have values between zero and one. One indicates that an event will definitely occur, while zero indicates that an event will not occur. Likewise, probabilities expressed as percentages possess values between zero and one hundred percent where probabilities closer to zero are unlikely to occur and those close to one hundred percent are more likely to occur.
Now that we understand the definition of a probability in its most general sense, we can investigate conditional probabilities. A conditional probability is defined as the probability that event B will occur given the information that an event A has already occurred.
It is expressed using the following equation:
In this equation, the probability of event B given that event A has occurred is equal to the probability of the intersection of events A and B divided by the probability of event A. It is important to note that if the events are independent, then the probability of event B given event A is simply the probability of event B because event A does not affect it.
Before we can use this information to discuss the general multiplication rule of probability, we must understand what is meant by the terms uniform and non-uniform in a probability model. In a uniform probability mode all events possess an equal chance of occurring. For instance, rolling any one side on a six sided fair die will have the same probability: one out of six. Likewise, the probability of a spinner landing on any of the colored spaces in the uniform figure is ten percent; therefore, uniform probability models posses events that all have the same probability of occurring.
On the other hand, in non-uniform models events do not share an equal probabilities. For instance, someone may weight or alter a die in order to increase the chances of rolling a particular number. Likewise, the probability of a spinner landing on each of the colored spaces in the non-uniform image possess differing probabilities; thus, the probabilities of non-uniform events differ from one another.
Now that we understand what is meant by uniform and non-uniform probability models, we can discuss the general multiplication role of probability. First, it can only be used to answer questions regarding uniform models because non-uniform models require more advanced mathematics. Second, the formula is based upon the conditional probability formula. Let's start by reviewing this formula.
Let's begin by multiplying each side of the equation by the following probability:
Cross out like terms and simplify in order to create our first rule of general multiplication of probabilities.
Next, we can use the same model to create another multiplication formula for the conditional probability:
Simplify as before in order to create our second rule of general multiplication of probabilities.
We can create a third rule of general multiplication of probabilities by setting the two equations equal to one another by using the like term:
These formulas enable us to easily solve probabilities problems that may be lacking information. Let's use this information to solve the problem. We need to find the probability of given the following information:
We will start by choosing the correct formula. In this case, we shall use the first formula:
Now, we can substitute in known values.
Solve.
Example Question #10 : General Multiplication Rule In A Uniform Probability Model: Ccss.Math.Content.Hss Cp.B.8
Use the general multiplication rule to solve for the given probability model. For events A and B, calculate P(A\cap B) given the following information:
Cannot be determined
In order to solve this problem, we need to discuss probabilities, conditional probabilities, and the general multiplication rule of probability. A probability is generally defined as the chances or likelihood of an event occurring. It is calculated by identifying two components: the event and the sample space. The event is defined as the favorable outcome or success that we wish to observe. On the other hand, the sample space is defined as the set of all possible outcomes for the event. Mathematically we calculate probabilities by dividing the event by the sample space:
Let's use a simple example: the rolling of a die. We want to know the probability of rolling a one. We know that the sample space is six because there are six sides or outcomes to the die. Also, we know that there is only a single side with a value of one; therefore,
Now, let's convert this into a percentage:
Probabilities expressed in fraction form will have values between zero and one. One indicates that an event will definitely occur, while zero indicates that an event will not occur. Likewise, probabilities expressed as percentages possess values between zero and one hundred percent where probabilities closer to zero are unlikely to occur and those close to one hundred percent are more likely to occur.
Now that we understand the definition of a probability in its most general sense, we can investigate conditional probabilities. A conditional probability is defined as the probability that event B will occur given the information that an event A has already occurred.
It is expressed using the following equation:
In this equation, the probability of event B given that event A has occurred is equal to the probability of the intersection of events A and B divided by the probability of event A. It is important to note that if the events are independent, then the probability of event B given event A is simply the probability of event B because event A does not affect it.
Before we can use this information to discuss the general multiplication rule of probability, we must understand what is meant by the terms uniform and non-uniform in a probability model. In a uniform probability mode all events possess an equal chance of occurring. For instance, rolling any one side on a six sided fair die will have the same probability: one out of six. Likewise, the probability of a spinner landing on any of the colored spaces in the uniform figure is ten percent; therefore, uniform probability models posses events that all have the same probability of occurring.
On the other hand, in non-uniform models events do not share an equal probabilities. For instance, someone may weight or alter a die in order to increase the chances of rolling a particular number. Likewise, the probability of a spinner landing on each of the colored spaces in the non-uniform image possess differing probabilities; thus, the probabilities of non-uniform events differ from one another.
Now that we understand what is meant by uniform and non-uniform probability models, we can discuss the general multiplication role of probability. First, it can only be used to answer questions regarding uniform models because non-uniform models require more advanced mathematics. Second, the formula is based upon the conditional probability formula. Let's start by reviewing this formula.
Let's begin by multiplying each side of the equation by the following probability:
Cross out like terms and simplify in order to create our first rule of general multiplication of probabilities.
Next, we can use the same model to create another multiplication formula for the conditional probability:
Simplify as before in order to create our second rule of general multiplication of probabilities.
We can create a third rule of general multiplication of probabilities by setting the two equations equal to one another by using the like term:
These formulas enable us to easily solve probabilities problems that may be lacking information. Let's use this information to solve the problem. We need to find the probability of given the following information:
We will start by choosing the correct formula. In this case, we shall use the first formula:
Now, we can substitute in known values.
Solve.
Example Question #11 : General Multiplication Rule In A Uniform Probability Model: Ccss.Math.Content.Hss Cp.B.8
Use the general multiplication rule to solve for the given probability model. For events A and B, calculate P(A\cap B) given the following information:
Cannot be determined
In order to solve this problem, we need to discuss probabilities, conditional probabilities, and the general multiplication rule of probability. A probability is generally defined as the chances or likelihood of an event occurring. It is calculated by identifying two components: the event and the sample space. The event is defined as the favorable outcome or success that we wish to observe. On the other hand, the sample space is defined as the set of all possible outcomes for the event. Mathematically we calculate probabilities by dividing the event by the sample space:
Let's use a simple example: the rolling of a die. We want to know the probability of rolling a one. We know that the sample space is six because there are six sides or outcomes to the die. Also, we know that there is only a single side with a value of one; therefore,
Now, let's convert this into a percentage:
Probabilities expressed in fraction form will have values between zero and one. One indicates that an event will definitely occur, while zero indicates that an event will not occur. Likewise, probabilities expressed as percentages possess values between zero and one hundred percent where probabilities closer to zero are unlikely to occur and those close to one hundred percent are more likely to occur.
Now that we understand the definition of a probability in its most general sense, we can investigate conditional probabilities. A conditional probability is defined as the probability that event B will occur given the information that an event A has already occurred.
It is expressed using the following equation:
In this equation, the probability of event B given that event A has occurred is equal to the probability of the intersection of events A and B divided by the probability of event A. It is important to note that if the events are independent, then the probability of event B given event A is simply the probability of event B because event A does not affect it.
Before we can use this information to discuss the general multiplication rule of probability, we must understand what is meant by the terms uniform and non-uniform in a probability model. In a uniform probability mode all events possess an equal chance of occurring. For instance, rolling any one side on a six sided fair die will have the same probability: one out of six. Likewise, the probability of a spinner landing on any of the colored spaces in the uniform figure is ten percent; therefore, uniform probability models posses events that all have the same probability of occurring.
On the other hand, in non-uniform models events do not share an equal probabilities. For instance, someone may weight or alter a die in order to increase the chances of rolling a particular number. Likewise, the probability of a spinner landing on each of the colored spaces in the non-uniform image possess differing probabilities; thus, the probabilities of non-uniform events differ from one another.
Now that we understand what is meant by uniform and non-uniform probability models, we can discuss the general multiplication role of probability. First, it can only be used to answer questions regarding uniform models because non-uniform models require more advanced mathematics. Second, the formula is based upon the conditional probability formula. Let's start by reviewing this formula.
Let's begin by multiplying each side of the equation by the following probability:
Cross out like terms and simplify in order to create our first rule of general multiplication of probabilities.
Next, we can use the same model to create another multiplication formula for the conditional probability:
Simplify as before in order to create our second rule of general multiplication of probabilities.
We can create a third rule of general multiplication of probabilities by setting the two equations equal to one another by using the like term:
These formulas enable us to easily solve probabilities problems that may be lacking information. Let's use this information to solve the problem. We need to find the probability of given the following information:
We will start by choosing the correct formula. In this case, we shall use the first formula:
Now, we can substitute in known values.
Solve.
Example Question #12 : General Multiplication Rule In A Uniform Probability Model: Ccss.Math.Content.Hss Cp.B.8
Use the general multiplication rule to solve for the given probability model. For events A and B, calculate P(A\cap B) given the following information:
Cannot be determined
In order to solve this problem, we need to discuss probabilities, conditional probabilities, and the general multiplication rule of probability. A probability is generally defined as the chances or likelihood of an event occurring. It is calculated by identifying two components: the event and the sample space. The event is defined as the favorable outcome or success that we wish to observe. On the other hand, the sample space is defined as the set of all possible outcomes for the event. Mathematically we calculate probabilities by dividing the event by the sample space:
Let's use a simple example: the rolling of a die. We want to know the probability of rolling a one. We know that the sample space is six because there are six sides or outcomes to the die. Also, we know that there is only a single side with a value of one; therefore,
Now, let's convert this into a percentage:
Probabilities expressed in fraction form will have values between zero and one. One indicates that an event will definitely occur, while zero indicates that an event will not occur. Likewise, probabilities expressed as percentages possess values between zero and one hundred percent where probabilities closer to zero are unlikely to occur and those close to one hundred percent are more likely to occur.
Now that we understand the definition of a probability in its most general sense, we can investigate conditional probabilities. A conditional probability is defined as the probability that event B will occur given the information that an event A has already occurred.
It is expressed using the following equation:
In this equation, the probability of event B given that event A has occurred is equal to the probability of the intersection of events A and B divided by the probability of event A. It is important to note that if the events are independent, then the probability of event B given event A is simply the probability of event B because event A does not affect it.
Before we can use this information to discuss the general multiplication rule of probability, we must understand what is meant by the terms uniform and non-uniform in a probability model. In a uniform probability mode all events possess an equal chance of occurring. For instance, rolling any one side on a six sided fair die will have the same probability: one out of six. Likewise, the probability of a spinner landing on any of the colored spaces in the uniform figure is ten percent; therefore, uniform probability models posses events that all have the same probability of occurring.
On the other hand, in non-uniform models events do not share an equal probabilities. For instance, someone may weight or alter a die in order to increase the chances of rolling a particular number. Likewise, the probability of a spinner landing on each of the colored spaces in the non-uniform image possess differing probabilities; thus, the probabilities of non-uniform events differ from one another.
Now that we understand what is meant by uniform and non-uniform probability models, we can discuss the general multiplication role of probability. First, it can only be used to answer questions regarding uniform models because non-uniform models require more advanced mathematics. Second, the formula is based upon the conditional probability formula. Let's start by reviewing this formula.
Let's begin by multiplying each side of the equation by the following probability:
Cross out like terms and simplify in order to create our first rule of general multiplication of probabilities.
Next, we can use the same model to create another multiplication formula for the conditional probability:
Simplify as before in order to create our second rule of general multiplication of probabilities.
We can create a third rule of general multiplication of probabilities by setting the two equations equal to one another by using the like term:
These formulas enable us to easily solve probabilities problems that may be lacking information. Let's use this information to solve the problem. We need to find the probability of given the following information:
We will start by choosing the correct formula. In this case, we shall use the first formula:
Now, we can substitute in known values.
Solve.
Example Question #13 : General Multiplication Rule In A Uniform Probability Model: Ccss.Math.Content.Hss Cp.B.8
Use the general multiplication rule to solve for the given probability model. For events A and B, calculate P(A\cap B) given the following information:
Cannot be determined
In order to solve this problem, we need to discuss probabilities, conditional probabilities, and the general multiplication rule of probability. A probability is generally defined as the chances or likelihood of an event occurring. It is calculated by identifying two components: the event and the sample space. The event is defined as the favorable outcome or success that we wish to observe. On the other hand, the sample space is defined as the set of all possible outcomes for the event. Mathematically we calculate probabilities by dividing the event by the sample space:
Let's use a simple example: the rolling of a die. We want to know the probability of rolling a one. We know that the sample space is six because there are six sides or outcomes to the die. Also, we know that there is only a single side with a value of one; therefore,
Now, let's convert this into a percentage:
Probabilities expressed in fraction form will have values between zero and one. One indicates that an event will definitely occur, while zero indicates that an event will not occur. Likewise, probabilities expressed as percentages possess values between zero and one hundred percent where probabilities closer to zero are unlikely to occur and those close to one hundred percent are more likely to occur.
Now that we understand the definition of a probability in its most general sense, we can investigate conditional probabilities. A conditional probability is defined as the probability that event B will occur given the information that an event A has already occurred.
It is expressed using the following equation:
In this equation, the probability of event B given that event A has occurred is equal to the probability of the intersection of events A and B divided by the probability of event A. It is important to note that if the events are independent, then the probability of event B given event A is simply the probability of event B because event A does not affect it.
Before we can use this information to discuss the general multiplication rule of probability, we must understand what is meant by the terms uniform and non-uniform in a probability model. In a uniform probability mode all events possess an equal chance of occurring. For instance, rolling any one side on a six sided fair die will have the same probability: one out of six. Likewise, the probability of a spinner landing on any of the colored spaces in the uniform figure is ten percent; therefore, uniform probability models posses events that all have the same probability of occurring.
On the other hand, in non-uniform models events do not share an equal probabilities. For instance, someone may weight or alter a die in order to increase the chances of rolling a particular number. Likewise, the probability of a spinner landing on each of the colored spaces in the non-uniform image possess differing probabilities; thus, the probabilities of non-uniform events differ from one another.
Now that we understand what is meant by uniform and non-uniform probability models, we can discuss the general multiplication role of probability. First, it can only be used to answer questions regarding uniform models because non-uniform models require more advanced mathematics. Second, the formula is based upon the conditional probability formula. Let's start by reviewing this formula.
Let's begin by multiplying each side of the equation by the following probability:
Cross out like terms and simplify in order to create our first rule of general multiplication of probabilities.
Next, we can use the same model to create another multiplication formula for the conditional probability:
Simplify as before in order to create our second rule of general multiplication of probabilities.
We can create a third rule of general multiplication of probabilities by setting the two equations equal to one another by using the like term:
These formulas enable us to easily solve probabilities problems that may be lacking information. Let's use this information to solve the problem. We need to find the probability of given the following information:
We will start by choosing the correct formula. In this case, we shall use the first formula:
Now, we can substitute in known values.
Solve.
Example Question #14 : General Multiplication Rule In A Uniform Probability Model: Ccss.Math.Content.Hss Cp.B.8
Use the general multiplication rule to solve for the given probability model. For events A and B, calculate P(A\cap B) given the following information:
Cannot be determined
In order to solve this problem, we need to discuss probabilities, conditional probabilities, and the general multiplication rule of probability. A probability is generally defined as the chances or likelihood of an event occurring. It is calculated by identifying two components: the event and the sample space. The event is defined as the favorable outcome or success that we wish to observe. On the other hand, the sample space is defined as the set of all possible outcomes for the event. Mathematically we calculate probabilities by dividing the event by the sample space:
Let's use a simple example: the rolling of a die. We want to know the probability of rolling a one. We know that the sample space is six because there are six sides or outcomes to the die. Also, we know that there is only a single side with a value of one; therefore,
Now, let's convert this into a percentage:
Probabilities expressed in fraction form will have values between zero and one. One indicates that an event will definitely occur, while zero indicates that an event will not occur. Likewise, probabilities expressed as percentages possess values between zero and one hundred percent where probabilities closer to zero are unlikely to occur and those close to one hundred percent are more likely to occur.
Now that we understand the definition of a probability in its most general sense, we can investigate conditional probabilities. A conditional probability is defined as the probability that event B will occur given the information that an event A has already occurred.
It is expressed using the following equation:
In this equation, the probability of event B given that event A has occurred is equal to the probability of the intersection of events A and B divided by the probability of event A. It is important to note that if the events are independent, then the probability of event B given event A is simply the probability of event B because event A does not affect it.
Before we can use this information to discuss the general multiplication rule of probability, we must understand what is meant by the terms uniform and non-uniform in a probability model. In a uniform probability mode all events possess an equal chance of occurring. For instance, rolling any one side on a six sided fair die will have the same probability: one out of six. Likewise, the probability of a spinner landing on any of the colored spaces in the uniform figure is ten percent; therefore, uniform probability models posses events that all have the same probability of occurring.
On the other hand, in non-uniform models events do not share an equal probabilities. For instance, someone may weight or alter a die in order to increase the chances of rolling a particular number. Likewise, the probability of a spinner landing on each of the colored spaces in the non-uniform image possess differing probabilities; thus, the probabilities of non-uniform events differ from one another.
Now that we understand what is meant by uniform and non-uniform probability models, we can discuss the general multiplication role of probability. First, it can only be used to answer questions regarding uniform models because non-uniform models require more advanced mathematics. Second, the formula is based upon the conditional probability formula. Let's start by reviewing this formula.
Let's begin by multiplying each side of the equation by the following probability:
Cross out like terms and simplify in order to create our first rule of general multiplication of probabilities.
Next, we can use the same model to create another multiplication formula for the conditional probability:
Simplify as before in order to create our second rule of general multiplication of probabilities.
We can create a third rule of general multiplication of probabilities by setting the two equations equal to one another by using the like term:
These formulas enable us to easily solve probabilities problems that may be lacking information. Let's use this information to solve the problem. We need to find the probability of given the following information:
We will start by choosing the correct formula. In this case, we shall use the first formula:
Now, we can substitute in known values.
Solve.
Example Question #1 : Permutations And Combinations Of Compound Events: Ccss.Math.Content.Hss Cp.B.9
The probability of winning a certain carnival game is . If there are six kids waiting in line to play the game, what is the probability that half of them will win the game?
.08
.01
.13
.7
.8
.13
Example Question #2 : Permutations And Combinations Of Compound Events: Ccss.Math.Content.Hss Cp.B.9
A researcher is studying the effects of hormone treatments on coleoptile and radicle growth in corn sprouts. The researcher wants to test the effects of four hormones: auxin, gibberellin, abscisic acid, and cytokinin. The researcher can only give each sprout a combination of three hormones and the order in which they are given matters for the study. How many outcomes are present in this study?
Cannot be determined
In order to solve this problem, we need to discuss probabilities, permutations, and combinations. A probability is generally defined as the chances or likelihood of an event occurring. It is calculated by identifying two components: the event and the sample space. The event is defined as the favorable outcome or success that we wish to observe. On the other hand, the sample space is defined as the set of all possible outcomes for the event. Mathematically we calculate probabilities by dividing the event by the sample space:
Let's use a simple example: the rolling of a die. We want to know the probability of rolling a one. We know that the sample space is six because there are six sides or outcomes to the die. Also, we know that there is only a single side with a value of one; therefore,
Now, let's convert this into a percentage:
Probabilities expressed in fraction form will have values between zero and one. One indicates that an event will definitely occur, while zero indicates that an event will not occur. Likewise, probabilities expressed as percentages possess values between zero and one hundred percent where probabilities closer to zero are unlikely to occur and those close to one hundred percent are more likely to occur.
But what happens if we do not know how many outcomes we have (e.g. in the question presented in the problem)? Outcomes are not always easily identified or calculated; however, mathematical operations associated with permutations and combinations can make these processes easier. Permutations provide the number of outcomes when the order of events matter. Permutations are calculated using the following formula:
In this formula, the variable, , refers to the number of things or items in the model and the variable,
, refers to the number of ways that items can be ordered (i.e. the number of bins or slots present in the model). Let's look at an example in order to better illustrate permutations. Suppose there are four different colored marbles—red, blue, white, and black— and a researcher wants to know how many outcomes are possible if a person picks two of the marbles when the order of the marbles matters. We know that this is a permutation because the order of the marbles matters. We need to assign numbers to each of the variables we have for items or marbles and we have two slots or bins that they are to be ordered into; therefore, we know the following:
Now, we need to calculate the number of permutations present in this model; however, we need to understand how to perform calculations involving factorials. Factorials are denoted with an exclamation point (!). For example, let's observe the following operation:
This denotes that for every non-negative integer, , we can define its factorial by calculating the product of all of the integers less than or equal to
.
Let's use this information to solve our marble example.
Solve.
Simplify.
We know that there are twelve possible permutations. We can write them in the following table:
Next, we need to discuss combinations. Combinations help us to calculate the number of outcomes in a given model when order does not matter. In other words, pulling a red and a blue marble is the same as pulling a blue and red marble. Combinations are calculated using the following formula:
The combination formula is similar to the permutation formula. In the combination formula the variable, , refers to the number of things or items in the model and the variable,
, refers to the number of ways that items can be ordered. Let's use the previous example to calculate the number of combinations in the model. Suppose there are four different colored marbles—red, blue, white, and black— and a researcher wants to know how many outcomes are possible if a person picks two of the marbles when the order of the marbles does not matter. In this model we can assume that the orders of the marbles pulled does not matter to the researcher. In cases where we do not care how combinations are ordered, we can use the combination formula.
Let's start by expanding the factorials.
Simplify.
Notice that the only difference between this and the permutation formula is that we have an additional term on the denominator where we have the factorial of the number of bins or slots multiplied by the factorial of the number of things minus the number of slots. We know that there are six different combinations of outcomes for this model. We can write them out in the following table:
Now, let's use this information to solve the given problem. We know that the researcher is testing four items or things—in this case the hormones: auxin, gibberellin, abscisic acid, and cytokinin. Next, we know that there we can have three hormones in each treatment or three slots. Last, the order of the hormones matters to the researcher. Given this information we must use the permutation formula.
Substitute in our known values.
Expand the factorials and solve.
There are twenty-four possible outcomes.
All Common Core: High School - Statistics and Probability Resources
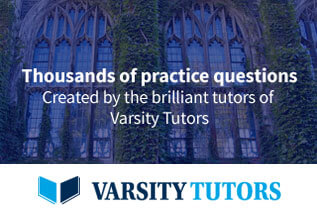