All Common Core: High School - Statistics and Probability Resources
Example Questions
Example Question #6 : Compute And Interpret The Correlation Coefficient Of A Linear Fit: Ccss.Math.Content.Hss Id.C.8
Which of the following graphs possesses a correlation coefficient indicative of a random distribution?
In order to solve this problem, we need to understand several key concepts associated with correlations. First, let's discuss what is meant by the term "correlation." A correlation exists when two variables possess a statistical relationship with one another. It is important to note that correlation in no way relates to causation. Causation implies that one variable causes change in the other, while correlation simply denotes the observation of a trend between two variables.
Second, let's observe the differences between slope and the correlation coefficient. The correlation coefficient is denoted by the following variable.
It is mathematically defined as a goodness of fit measure that is calculated by dividing the covariance of the samples by the product of the sample's standard deviations. This is also known as Pearson's r and it describes the strength and direction of a linear relationship between two variables. On the other hand, the slope is described as the gradient of a line and is key component of the slope intercept formula:
This formula provides information about two key parts of a line: the slope and y-intercept.
The slope is commonly defined as rise over run. In other words it is the change in y-values across points divided by the change in x-values. It is calculated using the following formula:
In this formula, the x and y-values come from two points from the line written in the following format:
It is important to note that slopes can be positive or negative. A positive slope moves upward from left to right while a negative slope moves downward. Even though the correlation coefficient will share the same sign as the slope, they mean entirely different things.
We have discussed the following distinctions: the differences between what is meant correlation and causation as well as the differences between the correlation coefficient and the slope. Now, we can start to solve the problem.
First, lets learn how calculate the correlation coefficient from coefficient of determination. The coefficient of determination is denoted by the following:
We can calculate the correlation coefficient by taking the square root of the coefficient of determination:
After we calculate the correlation coefficient, we need to know how to evaluate what the number means. We can pick the sign based on the position of the trendline or slope. If the slope is negative then the trendline travels downward from the left to the right of the graph. On the other hand, if the slope is positive then the trendline travels upwards from the left to the right side of the graph. Below is a table of values that explains the relationships between points based upon the correlation coefficient. A correlation coefficient close to zero indicates a random distribution.
We will start solving this problem by picking out the graph with obvious positive or negative trends and excluding them.
The last, two graph's have a near horizontal trendline, which is indicative of a random distribution:
This graph possesses a trendline with the following coefficient of determination:
We must take the square root of this measure calculated using statistical technology (this standard does not require you to calculate the correlation coefficient, only to interpret it).
This trendline's correlation coefficient is most indicative of a random distribution.
Example Question #181 : High School: Statistics & Probability
Which of the following graphs possesses a correlation coefficient indicative of a random distribution?
In order to solve this problem, we need to understand several key concepts associated with correlations. First, let's discuss what is meant by the term "correlation." A correlation exists when two variables possess a statistical relationship with one another. It is important to note that correlation in no way relates to causation. Causation implies that one variable causes change in the other, while correlation simply denotes the observation of a trend between two variables.
Second, let's observe the differences between slope and the correlation coefficient. The correlation coefficient is denoted by the following variable.
It is mathematically defined as a goodness of fit measure that is calculated by dividing the covariance of the samples by the product of the sample's standard deviations. This is also known as Pearson's r and it describes the strength and direction of a linear relationship between two variables. On the other hand, the slope is described as the gradient of a line and is key component of the slope intercept formula:
This formula provides information about two key parts of a line: the slope and y-intercept.
The slope is commonly defined as rise over run. In other words it is the change in y-values across points divided by the change in x-values. It is calculated using the following formula:
In this formula, the x and y-values come from two points from the line written in the following format:
It is important to note that slopes can be positive or negative. A positive slope moves upward from left to right while a negative slope moves downward. Even though the correlation coefficient will share the same sign as the slope, they mean entirely different things.
We have discussed the following distinctions: the differences between what is meant correlation and causation as well as the differences between the correlation coefficient and the slope. Now, we can start to solve the problem.
First, lets learn how calculate the correlation coefficient from coefficient of determination. The coefficient of determination is denoted by the following:
We can calculate the correlation coefficient by taking the square root of the coefficient of determination:
After we calculate the correlation coefficient, we need to know how to evaluate what the number means. We can pick the sign based on the position of the trendline or slope. If the slope is negative then the trendline travels downward from the left to the right of the graph. On the other hand, if the slope is positive then the trendline travels upwards from the left to the right side of the graph. Below is a table of values that explains the relationships between points based upon the correlation coefficient. A correlation coefficient close to zero indicates a random distribution.
We will start solving this problem by picking out the graph with obvious positive or negative trends and excluding them.
The last, two graph's have a near horizontal trendline, which is indicative of a random distribution:
This graph possesses a trendline with the following coefficient of determination:
We must take the square root of this measure calculated using statistical technology (this standard does not require you to calculate the correlation coefficient, only to interpret it).
This trendline's correlation coefficient is most indicative of a random distribution.
Example Question #182 : Interpreting Categorical & Quantitative Data
Which of the following graphs possesses a correlation coefficient indicative of a random distribution?
In order to solve this problem, we need to understand several key concepts associated with correlations. First, let's discuss what is meant by the term "correlation." A correlation exists when two variables possess a statistical relationship with one another. It is important to note that correlation in no way relates to causation. Causation implies that one variable causes change in the other, while correlation simply denotes the observation of a trend between two variables.
Second, let's observe the differences between slope and the correlation coefficient. The correlation coefficient is denoted by the following variable.
It is mathematically defined as a goodness of fit measure that is calculated by dividing the covariance of the samples by the product of the sample's standard deviations. This is also known as Pearson's r and it describes the strength and direction of a linear relationship between two variables. On the other hand, the slope is described as the gradient of a line and is key component of the slope intercept formula:
This formula provides information about two key parts of a line: the slope and y-intercept.
The slope is commonly defined as rise over run. In other words it is the change in y-values across points divided by the change in x-values. It is calculated using the following formula:
In this formula, the x and y-values come from two points from the line written in the following format:
It is important to note that slopes can be positive or negative. A positive slope moves upward from left to right while a negative slope moves downward. Even though the correlation coefficient will share the same sign as the slope, they mean entirely different things.
We have discussed the following distinctions: the differences between what is meant correlation and causation as well as the differences between the correlation coefficient and the slope. Now, we can start to solve the problem.
First, lets learn how calculate the correlation coefficient from coefficient of determination. The coefficient of determination is denoted by the following:
We can calculate the correlation coefficient by taking the square root of the coefficient of determination:
After we calculate the correlation coefficient, we need to know how to evaluate what the number means. We can pick the sign based on the position of the trendline or slope. If the slope is negative then the trendline travels downward from the left to the right of the graph. On the other hand, if the slope is positive then the trendline travels upwards from the left to the right side of the graph. Below is a table of values that explains the relationships between points based upon the correlation coefficient. A correlation coefficient close to zero indicates a random distribution.
We will start solving this problem by picking out the graph with obvious positive or negative trends and excluding them.
The last, two graph's have a near horizontal trendline, which is indicative of a random distribution:
This graph possesses a trendline with the following coefficient of determination:
We must take the square root of this measure calculated using statistical technology (this standard does not require you to calculate the correlation coefficient, only to interpret it).
This trendline's correlation coefficient is most indicative of a random distribution.
Example Question #183 : Interpreting Categorical & Quantitative Data
Which of the following graphs possesses a correlation coefficient indicative of a random distribution?
In order to solve this problem, we need to understand several key concepts associated with correlations. First, let's discuss what is meant by the term "correlation." A correlation exists when two variables possess a statistical relationship with one another. It is important to note that correlation in no way relates to causation. Causation implies that one variable causes change in the other, while correlation simply denotes the observation of a trend between two variables.
Second, let's observe the differences between slope and the correlation coefficient. The correlation coefficient is denoted by the following variable.
It is mathematically defined as a goodness of fit measure that is calculated by dividing the covariance of the samples by the product of the sample's standard deviations. This is also known as Pearson's r and it describes the strength and direction of a linear relationship between two variables. On the other hand, the slope is described as the gradient of a line and is key component of the slope intercept formula:
This formula provides information about two key parts of a line: the slope and y-intercept.
The slope is commonly defined as rise over run. In other words it is the change in y-values across points divided by the change in x-values. It is calculated using the following formula:
In this formula, the x and y-values come from two points from the line written in the following format:
It is important to note that slopes can be positive or negative. A positive slope moves upward from left to right while a negative slope moves downward. Even though the correlation coefficient will share the same sign as the slope, they mean entirely different things.
We have discussed the following distinctions: the differences between what is meant correlation and causation as well as the differences between the correlation coefficient and the slope. Now, we can start to solve the problem.
First, lets learn how calculate the correlation coefficient from coefficient of determination. The coefficient of determination is denoted by the following:
We can calculate the correlation coefficient by taking the square root of the coefficient of determination:
After we calculate the correlation coefficient, we need to know how to evaluate what the number means. We can pick the sign based on the position of the trendline or slope. If the slope is negative then the trendline travels downward from the left to the right of the graph. On the other hand, if the slope is positive then the trendline travels upwards from the left to the right side of the graph. Below is a table of values that explains the relationships between points based upon the correlation coefficient. A correlation coefficient close to zero indicates a random distribution.
We will start solving this problem by picking out the graph with obvious positive or negative trends and excluding them.
The last, two graph's have a near horizontal trendline, which is indicative of a random distribution:
This graph possesses a trendline with the following coefficient of determination:
We must take the square root of this measure calculated using statistical technology (this standard does not require you to calculate the correlation coefficient, only to interpret it).
This trendline's correlation coefficient is most indicative of a random distribution.
Example Question #181 : Interpreting Categorical & Quantitative Data
Violent crime has a strong positive correlation with ice cream sales. What can be inferred from this?
The correlation between ice cream sales and violent crime is the result of some error in the statistical study.
The increase in violent crime causes the increase in ice cream sales.
While the two events are correlated, more evidence is needed to determine whether or not this correlation is coincidental.
The two events are causally related.
The increase in ice cream sales causes the increase in violent crime.
While the two events are correlated, more evidence is needed to determine whether or not this correlation is coincidental.
Example Question #1 : Correlation Vs. Causation: Ccss.Math.Content.Hss Id.C.9
Which choice best describes the relationship between the variables in the following scatterplot?
None of these
Shark attacks induce ice cream consumption
The variables possess a correlation due to a lurking or linking variable
Ice cream consumption causes shark attacks
The variables possess a correlation due to a lurking or linking variable
In order to properly solve this question, we need to understand the differences between what is meant by correlation and causation. A correlation refers to the strength of the linear association between two quantitative variables. On the other hand, causation indicates that the change in one variable is the cause of change in another.
Correlation can be used as an indicator of causal relationships; however, experimentation is needed to properly identify which variable is actually causing the observed change. Scientific experimentation identifies causality through he implementation of laboratory procedures in a controlled setting. When variables are controlled, causation can be determined through observation and repeated tests.
Several logical fallacies explain why correlation does not directly imply causation. First, cause-and-effect is not determined by two events occurring simultaneously. In other words, events that occur together do not necessarily cause one another. Second, causality is not determined by an event preceding another temporally. In other words, this means that event B is not always a consequence of event A simply because event A occurs before event B.
Lurking or linking variables can cause events that are highly correlated to one another appear to have a casual relationship. This is because a third separate factor may be inducing change in the two variables.
Now, let's solve this problem. It asks us to describe the relationship in the scatterplot. We know that there is a positive relationship between the two variables; however, if we think critically we know that shark attacks and ice cream sales are independent of one another. The answers that suggest causality are incorrect. A linking or lurking variable—in this case warm temperatures—is causing change in both of the variables. In other words, warmer temperatures cause individuals to purchase ice cream and frequent the beach. Greater populations of beach goers increase the probability of shark attacks.
Example Question #2 : Correlation Vs. Causation: Ccss.Math.Content.Hss Id.C.9
Which choice best describes the relationship between the variables in the following scatterplot?
Beach attendance causes shark attacks
Shark attacks cause beach attendance
Beach attendance is negatively correlated with shark attacks
Beach attendance is positively correlated with shark attacks
Beach attendance is positively correlated with shark attacks
In order to properly solve this question, we need to understand the differences between what is meant by correlation and causation. A correlation refers to the strength of the linear association between two quantitative variables. On the other hand, causation indicates that the change in one variable is the cause of change in another.
Correlation can be used as an indicator of causal relationships; however, experimentation is needed to properly identify which variable is actually causing the observed change. Scientific experimentation identifies causality through he implementation of laboratory procedures in a controlled setting. When variables are controlled, causation can be determined through observation and repeated tests.
Several logical fallacies explain why correlation does not directly imply causation. First, cause-and-effect is not determined by two events occurring simultaneously. In other words, events that occur together do not necessarily cause one another. Second, causality is not determined by an event preceding another temporally. In other words, this means that event B is not always a consequence of event A simply because event A occurs before event B.
Lurking or linking variables can cause events that are highly correlated to one another appear to have a casual relationship. This is because a third separate factor may be inducing change in the two variables.
Now, let's solve this problem. It asks us to describe the relationship in the scatterplot. We know that there is a positive relationship between the two variables; however, if we think critically we know that beach attendance and shark attacks do not cause one another. The answers that suggest causality are incorrect. There are many factors that influence shark attacks on beaches—beach attendance is one of them. For example, if no one goes to the beach, then a shark located at the beach can attack no one. Increased beach attendance is positively correlated with shark attacks but further investigation is needed to determine if this causes the attacks. A mating cycle, global warming, or changes in food sources could all induce a shark attack. Beach attendance is only one factor correlated with this phenomenon.
Example Question #1 : Making Inferences & Justifying Conclusions
A car designer wants to know if customers prefer automatic or manual transmissions in cars. The designer hires a market research team to randomly sample and survey the preferences of potential car buyers in three major cities: New York, Chicago, and Los Angeles.
The data collected in this survey would be best described as which of the following?
None of these
Sample statistic
Population parameter
Population statistic
Sample parameter
Sample statistic
Solving questions related to this standard requires an understanding of definitions common to statistics. Specifically, this question is testing your knowledge of the difference between two fundamental statistical concepts: sample statistics and population parameters. Let's begin by discussing the differences between these two measures. Later, we will use this information to solve the problem.
First let's discuss what is meant by the term population. In statistics, a "population" is described as the entire group that is to be studied. An example of a population in the natural sciences would be every giant panda of the species Ailuropoda melanoleuca in the wild (1864 individuals according to the World Wildlife Foundation)—not captivity. Now, let's identify what is meant by the term population parameter. A "population parameter" is a statistic that is found by sampling the entire population. For example, the mean weight of the entire wild population of giant pandas in the world would be an example of a population parameter (i.e. the mean weight of all 1864 pandas). Next, we will discuss sample populations and statistics.
A "sample" is the subset of a population that is being studied. For example, researchers for a university want to study giant pandas in the wild but can only access a group of 100 pandas sampled in Sichuan, China. Data collected from this particular study would be known as a sample statistic (e.g. the mean weight of pandas in the Sichuan region). It is important to note that the external validity of some sample statistics are hindered. The external validity of a statistic is its ability to be applied to other samples and remain valid. If locals fed pandas in the Sichuan region, then their mean weight may be greater than those of the southern or northern regions. In this instance, the mean would not be representative of other populations of giant pandas.
Last, we should note that certain sample populations are better than others at predicting population parameters. A population parameter can be considered to be the true statistic of a given population while a sample statistic is only an estimate of a part or subset of the population. Simple random samples are good predictors of population parameters and can be used to estimate them. They are collected when every member in a population has an equal chance of being chosen (e.g. randomly selecting 100 of the 1864 pandas in the world).
Now, let's use this information to solve the question. The designer wants to know the preferences of potential car buyers; however, he only samples three major US cities. The data collected from this survey is an example of a sample statistic. It did not gather information from all of the potential car buyers for the particular company; therefore, the best answer is "sample statistic."
Example Question #2 : Making Inferences & Justifying Conclusions
Two college students, Joe and Melissa, are playing a tabletop role-playing game where snake eyes (a value of one on each of the two dice) allows one opponent to effectively attack the other. After three turns, Joe roles snake eyes three times consecutively while Melissa has not rolled it once. She begins to believe that Joe is using loaded dice, which would give him an unfair advantage. She decides to test this theory by rolling her fair dice three times in a row for sixty trials. Melissa knows that the probability of rolling snake eyes is fairly low; furthermore, after sixty trials she only roles snake eyes two times in a row.
Which of the following will Melissa most likely conclude?
Melissa miscalculated the probability of rolling snake eyes
Melissa cannot tell if Joe is using loaded or fair dice
Joe has tricked her by using loaded dice
Joe is using fair dice
Joe has tricked her by using loaded dice
This question is asking us to use a simulation in order to determine whether or not an observed phenomenon is statistically probable. We will do this by creating and testing a hypothesis. Afterwards, we can use our collected data to make a conclusion as to whether or not Joe is using fair dice in this scenario.
Hypotheses are "if/then" statements that represent an inference or educated guess regarding a particular phenomenon. They are tested through experimentation. The results of an experiment will reveal if a hypothesis can be supported or not. At this point, it is important tot note that a hypothesis can never be proven: experimentation can only support or refute a hypothesis. Even scientific theories cannot be proven they only have a mass of supporting studies to add to their scientific validity.
Before we solve this problem, we should review the scientific process. In the scientific method we observe a phenomenon, gather background information, develop a tentative explanation (i.e. a hypothesis), test this explanation through the observation and manipulation of variables, and, finally, we create conclusions based upon experimentation. These conclusions will either support or refute the hypothesis.
Now, let's use this information to solve the problem regarding whether or not Joe is using fair dice. In this problem, Melissa noticed that Joe rolled snake eyes three times in a row. She gathered background information and identified the following probability calculations:
From this information, she realized that the probability of rolling snake eyes three times in a row is very low. Using this information, Melissa created an experiment, In this experiment, she rolled her known fair dice three times in a row for sixty trials. In these sixty trials she was only able to roll snake eyes in a row two times. From this information she was able to make the following conclusion: "Joe has tricked her by using loaded dice."
In this lesson we have learned how to use simulations and the scientific method in order to determine whether or not an event is the product of random chance or manipulation (i.e. Joe tricking Melissa with loaded dice).
Example Question #1 : Making Inferences & Justifying Conclusions
Two college students, Joe and Melissa, are playing a tabletop role-playing game where snake eyes (a value of one on each of the two dice) allows one opponent to effectively attack the other. After three turns, Joe roles snake eyes three times while Melissa has not rolled it once. She begins to believe that Joe is using loaded dice, which would give him an unfair advantage. She decides to test this theory by rolling her fair dice three times in a row for sixty trials. Melissa knows that the probability of rolling snake eyes is fairly low; furthermore, after sixty trials she only roles snake eyes two times in a row.
Which of the following will Melissa most likely conclude?
Joe is using fair dice
Melissa miscalculated the probability of rolling snake eyes
Melissa cannot tell if Joe is using loaded or fair dice
Joe has tricked her by using loaded dice
Joe has tricked her by using loaded dice
This question is asking us to use a simulation in order to determine whether or not an observed phenomenon is statistically probable. We will do this by creating and testing a hypothesis. Afterwards, we can use our collected data to make a conclusion as to whether or not Joe is using fair dice in this scenario.
Hypotheses are "if/then" statements that represent an inference or educated guess regarding a particular phenomenon. They are tested through experimentation. The results of an experiment will reveal if a hypothesis can be supported or not. At this point, it is important tot note that a hypothesis can never be proven: experimentation can only support or refute a hypothesis. Even scientific theories cannot be proven they only have a mass of supporting studies to add to their scientific validity.
Before we solve this problem, we should review the scientific process. In the scientific method we observe a phenomenon, gather background information, develop a tentative explanation (i.e. a hypothesis), test this explanation through the observation and manipulation of variables, and, finally, we create conclusions based upon experimentation. These conclusions will either support or refute the hypothesis.
Now, let's use this information to solve the problem regarding whether or not Joe is using fair dice. In this problem, Melissa noticed that Joe rolled snake eyes three times in a row. She gathered background information and identified the following probability calculations:
From this information, she realized that the probability of rolling snake eyes three times in a row is very low. Using this information, Melissa created an experiment, In this experiment, she rolled her known fair dice three times in a row for sixty trials. In these sixty trials she was only able to roll snake eyes in a row two times. From this information she was able to make the following conclusion: "Joe has tricked her by using loaded dice."
In this lesson we have learned how to use simulations and the scientific method in order to determine whether or not an event is the product of random chance or manipulation (i.e. Joe tricking Melissa with loaded dice).
Certified Tutor
Certified Tutor
All Common Core: High School - Statistics and Probability Resources
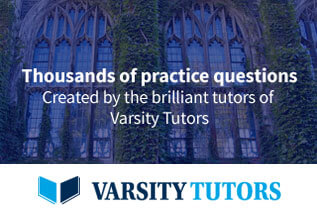