All Common Core: High School - Statistics and Probability Resources
Example Questions
Example Question #211 : High School: Statistics & Probability
A car manufacturer wants to produce a sports car that has an average quarter mile run time of:
Researchers decide to randomly sample two hundred cars off of the stock production line. They find that these cars' mean quarter mile run time is as follows:
Is it reasonable to say that the car performs to the manufacturer's specifications?
Cannot be determined
No
Yes
Yes
In order to solve this question, we need to learn how to infer population parameters from sample statistics. We will do this by reviewing the definitions of parameters and statistics, recalling the processes associated with the scientific method, and by interrogating the information in the problem.
First, let's discuss what is meant by the term population. In statistics, a "population" is described as the entire group that is to be studied. An example of a population in the natural sciences would be every giant panda of the species Ailuropoda melanoleuca in the wild (1864 individuals according to the World Wildlife Foundation)—not captivity. Now, let's identify what is meant by the term population parameter. A "population parameter" is a statistic that is found by sampling the entire population. For example, the mean weight of the entire wild population of giant pandas in the world would be an example of a population parameter (i.e. the mean weight of all 1864 pandas). Next, we will discuss sample populations and statistics.
A "sample" is the subset of a population that is being studied. For example, researchers for a university want to study giant pandas in the wild but can only access a group of 100 pandas sampled in Sichuan, China. Data collected from this particular study would be known as a sample statistic (e.g. the mean weight of pandas in the Sichuan region). It is important to note that the external validity of some sample statistics are hindered. The external validity of a statistic is its ability to be applied to other samples and remain valid. If locals fed pandas in the Sichuan region, then their mean weight may be greater than those of the southern or northern regions. In this instance, the mean would not be representative of other populations of giant pandas.
Last, we should note that certain sample populations are better than others at predicting population parameters. A population parameter can be considered to be the true statistic of a given population while a sample statistic is only an estimate of a part or subset of the population. Simple random samples are good predictors of population parameters and can be used to estimate them. They are collected when every member in a population has an equal chance of being chosen (e.g. randomly selecting 100 of the 1864 pandas in the world).
Next, we shall observe the steps associated with the scientific method.
In this method, researchers observe a phenomenon and develop a tentative explanation for it. Afterwards, they develop an experiment, which assigns subjects or variables to control and treatment groups. Experiments measure the change that an independent variable (i.e. treatment) has on a dependent variable (i.e. subject or phenomena under investigation). Scientists repeat or replicate these experiments multiple times in order gather data for statistical analysis. Scientists analyze the data and then use this evidence to either support or refute a hypothesis. Hypotheses can only be supported or refuted: never proven. This is because scientific investigation can only gather evidence for or against a particular phenomenon. Even theories such as gravity and natural selection have not been proven—they are simply supported by an almost endless amount of scientific evidence.
Last, let's use this information to solve the following problem:
A car manufacturer wants to produce a sports car that has the following average quarter mile run time:
Researchers decide to randomly sample two hundred cars off of the stock production line. They find that these cars' quarter mile run mean time is as follows:
Is it reasonable to say that the car performs to the manufacturer's specifications?
The researchers found that the mean time of two hundred cars was:
According to this sample statistic, it is reasonable to assume that the car can and will perform to the specifications of the manufacturer. This is because the calculated mean run time is only slightly below the desired average quarter mile run time. The time that was calculated in the experiment represents the test statistic, while the manufacturer's desired quarter mile run time represents the population parameter. The statistic represents a single experiment used to test a hypothesis that stated: the new car has an average quarter mile run time of
This hypothesis was tested by a single experiment that measured the quarter mile run time of two hundred stock cars. Replication of this experiment would gather means of multiple samples. If the researchers were able to gather a census of the quarter mile run time of the population, then they could create a standard curve:
A standard distribution represents a distribution of the means; furthermore, its mean (i.e. the mean of the means) is hypothetically equal to the population parameter mean.
It is costly and unlikely that a researcher will be able to take a census of all the instances of a particular phenomenon. As a result, many researchers will take a limited sample and fit a standard curve to it in order to gather a representative estimate of a populations mean—in this case quarter mile run times. In this example, researchers could gather the mean of one hundred experiments and create the following graph:
We can see in this graph that the sample statistic hovers around the center—mean of the means—of the graph; therefore, it is reasonable to assume that this statistic supports the hypothesis that stated that the car could meet designer specifications.
Example Question #212 : High School: Statistics & Probability
A car manufacturer wants to produce a sports car that has an average quarter mile run time of:
Researchers decide to randomly sample two hundred cars off of the stock production line. They find that these cars' mean quarter mile run time is as follows:
Is it reasonable to say that the car performs to the manufacturer's specifications?
No
Yes
Cannot be determined
No
In order to solve this question, we need to learn how to infer population parameters from sample statistics. We will do this by reviewing the definitions of parameters and statistics, recalling the processes associated with the scientific method, and by interrogating the information in the problem.
First, let's discuss what is meant by the term population. In statistics, a "population" is described as the entire group that is to be studied. An example of a population in the natural sciences would be every giant panda of the species Ailuropoda melanoleuca in the wild (1864 individuals according to the World Wildlife Foundation)—not captivity. Now, let's identify what is meant by the term population parameter. A "population parameter" is a statistic that is found by sampling the entire population. For example, the mean weight of the entire wild population of giant pandas in the world would be an example of a population parameter (i.e. the mean weight of all 1864 pandas). Next, we will discuss sample populations and statistics.
A "sample" is the subset of a population that is being studied. For example, researchers for a university want to study giant pandas in the wild but can only access a group of 100 pandas sampled in Sichuan, China. Data collected from this particular study would be known as a sample statistic (e.g. the mean weight of pandas in the Sichuan region). It is important to note that the external validity of some sample statistics are hindered. The external validity of a statistic is its ability to be applied to other samples and remain valid. If locals fed pandas in the Sichuan region, then their mean weight may be greater than those of the southern or northern regions. In this instance, the mean would not be representative of other populations of giant pandas.
Last, we should note that certain sample populations are better than others at predicting population parameters. A population parameter can be considered to be the true statistic of a given population while a sample statistic is only an estimate of a part or subset of the population. Simple random samples are good predictors of population parameters and can be used to estimate them. They are collected when every member in a population has an equal chance of being chosen (e.g. randomly selecting 100 of the 1864 pandas in the world).
Next, we shall observe the steps associated with the scientific method.
In this method, researchers observe a phenomenon and develop a tentative explanation for it. Afterwards, they develop an experiment, which assigns subjects or variables to control and treatment groups. Experiments measure the change that an independent variable (i.e. treatment) has on a dependent variable (i.e. subject or phenomena under investigation). Scientists repeat or replicate these experiments multiple times in order gather data for statistical analysis. Scientists analyze the data and then use this evidence to either support or refute a hypothesis. Hypotheses can only be supported or refuted: never proven. This is because scientific investigation can only gather evidence for or against a particular phenomenon. Even theories such as gravity and natural selection have not been proven—they are simply supported by an almost endless amount of scientific evidence.
Last, let's use this information to solve the following problem:
A car manufacturer wants to produce a sports car that has the following average quarter mile run time:
Researchers decide to randomly sample two hundred cars off of the stock production line. They find that these cars' quarter mile run mean time is as follows:
Is it reasonable to say that the car performs to the manufacturer's specifications?
The researchers found that the mean time of two hundred cars was:
According to this sample statistic, it is not reasonable to assume that the car can perform to the specifications of the manufacturer. This is because the calculated mean run time is far above the desired average quarter mile run time. The time that was calculated in the experiment represents the test statistic, while the manufacturer's desired quarter mile run time represents the population parameter. The statistic represents a single experiment used to test a hypothesis that stated: the new car has an average quarter mile run time of
This hypothesis was tested by a single experiment that measured the quarter mile run time of two hundred stock cars. Replication of this experiment would gather means of multiple samples. If the researchers were able to gather a census of the quarter mile run time of the population, then they could create a standard curve:
A standard distribution represents a distribution of the means; furthermore, its mean (i.e. the mean of the means) is hypothetically equal to the population parameter mean.
It is costly and unlikely that a researcher will be able to take a census of all the instances of a particular phenomenon. As a result, many researchers will take a limited sample and fit a standard curve to it in order to gather a representative estimate of a populations mean—in this case quarter mile run times. In this example, researchers could gather the mean of one hundred experiments and create the following graph:
We can see in this graph that the sample statistic doesn't hover around the center—mean of the means—of the graph; therefore, it is not reasonable to assume that this statistic supports the hypothesis that stated that the car could meet designer specifications.
Example Question #211 : High School: Statistics & Probability
A car manufacturer wants to produce a sports car that has an average quarter mile run time of:
Researchers decide to randomly sample two hundred cars off of the stock production line. They find that these cars' mean quarter mile run time is as follows:
Is it reasonable to say that the car performs to the manufacturer's specifications?
Cannot be determined
No
Yes
No
In order to solve this question, we need to learn how to infer population parameters from sample statistics. We will do this by reviewing the definitions of parameters and statistics, recalling the processes associated with the scientific method, and by interrogating the information in the problem.
First, let's discuss what is meant by the term population. In statistics, a "population" is described as the entire group that is to be studied. An example of a population in the natural sciences would be every giant panda of the species Ailuropoda melanoleuca in the wild (1864 individuals according to the World Wildlife Foundation)—not captivity. Now, let's identify what is meant by the term population parameter. A "population parameter" is a statistic that is found by sampling the entire population. For example, the mean weight of the entire wild population of giant pandas in the world would be an example of a population parameter (i.e. the mean weight of all 1864 pandas). Next, we will discuss sample populations and statistics.
A "sample" is the subset of a population that is being studied. For example, researchers for a university want to study giant pandas in the wild but can only access a group of 100 pandas sampled in Sichuan, China. Data collected from this particular study would be known as a sample statistic (e.g. the mean weight of pandas in the Sichuan region). It is important to note that the external validity of some sample statistics are hindered. The external validity of a statistic is its ability to be applied to other samples and remain valid. If locals fed pandas in the Sichuan region, then their mean weight may be greater than those of the southern or northern regions. In this instance, the mean would not be representative of other populations of giant pandas.
Last, we should note that certain sample populations are better than others at predicting population parameters. A population parameter can be considered to be the true statistic of a given population while a sample statistic is only an estimate of a part or subset of the population. Simple random samples are good predictors of population parameters and can be used to estimate them. They are collected when every member in a population has an equal chance of being chosen (e.g. randomly selecting 100 of the 1864 pandas in the world).
Next, we shall observe the steps associated with the scientific method.
In this method, researchers observe a phenomenon and develop a tentative explanation for it. Afterwards, they develop an experiment, which assigns subjects or variables to control and treatment groups. Experiments measure the change that an independent variable (i.e. treatment) has on a dependent variable (i.e. subject or phenomena under investigation). Scientists repeat or replicate these experiments multiple times in order gather data for statistical analysis. Scientists analyze the data and then use this evidence to either support or refute a hypothesis. Hypotheses can only be supported or refuted: never proven. This is because scientific investigation can only gather evidence for or against a particular phenomenon. Even theories such as gravity and natural selection have not been proven—they are simply supported by an almost endless amount of scientific evidence.
Last, let's use this information to solve the following problem:
A car manufacturer wants to produce a sports car that has the following average quarter mile run time:
Researchers decide to randomly sample two hundred cars off of the stock production line. They find that these cars' quarter mile run mean time is as follows:
Is it reasonable to say that the car performs to the manufacturer's specifications?
The researchers found that the mean time of two hundred cars was:
According to this sample statistic, it is not reasonable to assume that the car can perform to the specifications of the manufacturer. This is because the calculated mean run time is far above the desired average quarter mile run time. The time that was calculated in the experiment represents the test statistic, while the manufacturer's desired quarter mile run time represents the population parameter. The statistic represents a single experiment used to test a hypothesis that stated: the new car has an average quarter mile run time of
This hypothesis was tested by a single experiment that measured the quarter mile run time of two hundred stock cars. Replication of this experiment would gather means of multiple samples. If the researchers were able to gather a census of the quarter mile run time of the population, then they could create a standard curve:
A standard distribution represents a distribution of the means; furthermore, its mean (i.e. the mean of the means) is hypothetically equal to the population parameter mean.
It is costly and unlikely that a researcher will be able to take a census of all the instances of a particular phenomenon. As a result, many researchers will take a limited sample and fit a standard curve to it in order to gather a representative estimate of a populations mean—in this case quarter mile run times. In this example, researchers could gather the mean of one hundred experiments and create the following graph:
We can see in this graph that the sample statistic doesn't hover around the center—mean of the means—of the graph; therefore, it is not reasonable to assume that this statistic supports the hypothesis that stated that the car could meet designer specifications.
Example Question #212 : High School: Statistics & Probability
A car manufacturer wants to produce a sports car that has an average quarter mile run time of:
Researchers decide to randomly sample two hundred cars off of the stock production line. They find that these cars' mean quarter mile run time is as follows:
Is it reasonable to say that the car performs to the manufacturer's specifications?
Yes
Cannot be determined
No
Yes
In order to solve this question, we need to learn how to infer population parameters from sample statistics. We will do this by reviewing the definitions of parameters and statistics, recalling the processes associated with the scientific method, and by interrogating the information in the problem.
First, let's discuss what is meant by the term population. In statistics, a "population" is described as the entire group that is to be studied. An example of a population in the natural sciences would be every giant panda of the species Ailuropoda melanoleuca in the wild (1864 individuals according to the World Wildlife Foundation)—not captivity. Now, let's identify what is meant by the term population parameter. A "population parameter" is a statistic that is found by sampling the entire population. For example, the mean weight of the entire wild population of giant pandas in the world would be an example of a population parameter (i.e. the mean weight of all 1864 pandas). Next, we will discuss sample populations and statistics.
A "sample" is the subset of a population that is being studied. For example, researchers for a university want to study giant pandas in the wild but can only access a group of 100 pandas sampled in Sichuan, China. Data collected from this particular study would be known as a sample statistic (e.g. the mean weight of pandas in the Sichuan region). It is important to note that the external validity of some sample statistics are hindered. The external validity of a statistic is its ability to be applied to other samples and remain valid. If locals fed pandas in the Sichuan region, then their mean weight may be greater than those of the southern or northern regions. In this instance, the mean would not be representative of other populations of giant pandas.
Last, we should note that certain sample populations are better than others at predicting population parameters. A population parameter can be considered to be the true statistic of a given population while a sample statistic is only an estimate of a part or subset of the population. Simple random samples are good predictors of population parameters and can be used to estimate them. They are collected when every member in a population has an equal chance of being chosen (e.g. randomly selecting 100 of the 1864 pandas in the world).
Next, we shall observe the steps associated with the scientific method.
In this method, researchers observe a phenomenon and develop a tentative explanation for it. Afterwards, they develop an experiment, which assigns subjects or variables to control and treatment groups. Experiments measure the change that an independent variable (i.e. treatment) has on a dependent variable (i.e. subject or phenomena under investigation). Scientists repeat or replicate these experiments multiple times in order gather data for statistical analysis. Scientists analyze the data and then use this evidence to either support or refute a hypothesis. Hypotheses can only be supported or refuted: never proven. This is because scientific investigation can only gather evidence for or against a particular phenomenon. Even theories such as gravity and natural selection have not been proven—they are simply supported by an almost endless amount of scientific evidence.
Last, let's use this information to solve the following problem:
A car manufacturer wants to produce a sports car that has the following average quarter mile run time:
Researchers decide to randomly sample two hundred cars off of the stock production line. They find that these cars' quarter mile run mean time is as follows:
Is it reasonable to say that the car performs to the manufacturer's specifications?
The researchers found that the mean time of two hundred cars was:
According to this sample statistic, it is reasonable to assume that the car can and will perform to the specifications of the manufacturer. This is because the calculated mean run time is only slightly below the desired average quarter mile run time. The time that was calculated in the experiment represents the test statistic, while the manufacturer's desired quarter mile run time represents the population parameter. The statistic represents a single experiment used to test a hypothesis that stated: the new car has an average quarter mile run time of
This hypothesis was tested by a single experiment that measured the quarter mile run time of two hundred stock cars. Replication of this experiment would gather means of multiple samples. If the researchers were able to gather a census of the quarter mile run time of the population, then they could create a standard curve:
A standard distribution represents a distribution of the means; furthermore, its mean (i.e. the mean of the means) is hypothetically equal to the population parameter mean.
It is costly and unlikely that a researcher will be able to take a census of all the instances of a particular phenomenon. As a result, many researchers will take a limited sample and fit a standard curve to it in order to gather a representative estimate of a populations mean—in this case quarter mile run times. In this example, researchers could gather the mean of one hundred experiments and create the following graph:
We can see in this graph that the sample statistic hovers around the center—mean of the means—of the graph; therefore, it is reasonable to assume that this statistic supports the hypothesis that stated that the car could meet designer specifications.
Example Question #213 : High School: Statistics & Probability
A group of scientists studied the effects of hormone treatments on plant germination. They decided to study Zea mays or corn. They hypothesize that abscisic acid will inhibit plant growth, while gibberellins will counteract this germination inhibition. Scientists leave some seeds unaltered (i.e. the control group) and treat one group of seeds with abscisic acid, while a third group is treated with both abscisic acid and gibberellins. They continue these treatments for fourteen days and monitored the plant's growth. The data of this study is located in the provided figure.
Was there an observable difference between treatments in the study?
No, abscisic acid promotes plant growth and gibberellins can counteract growth promotion
Yes, gibberellins can counteract abscisic acid inhibition
No, abscisic acid inhibits plant growth
Yes, abscisic acid inhibits plant growth and gibberellins can counteract growth inhibition
Yes, abscisic acid promotes plant growth
Yes, abscisic acid inhibits plant growth and gibberellins can counteract growth inhibition
In order to solve this problem, let's first observe the steps associated with the scientific method. In this particular experiment we can see that the scientists have interest in a particular biological phenomenon: the effects of hormones on plant growth and germination. They develop a hypothesis based on these observations. A hypothesis is a tentative explanation for an observed phenomenon. In this experiment the scientists developed the following hypothesis: abscisic acid will inhibit plant growth, while gibberellins will counteract this germination inhibition. A null hypothesis is a statement of no difference. In other words, plant hormones will have no effect on plant growth.
In order to support or refute this hypothesis, the scientists developed a simulation or experiment. In this scenario, they exposed corn seeds to differing hormone treatments:
- No treatment
- Abscisic acid
- Gibberellins and abscisic acid
They monitored the seeds for fourteen days and created a box plot using the data.
Let's look at how box plots are used to analyze data.
Box plots are broken into five main parts that create four primary regions. Each region contains a quarter of the data in the plot. The plot is initially broken into two regions using the following values: the minimum value, the maximum value, and the median or average of the set. Next, the minimum and median values are divided by the median of the lower half of the data or the first/lower quartile. Likewise, the median and the maximum values are divided by the median of the upper half of the data set or third/upper quartile. If the box is bigger, then there is a greater variance or spread in the data. Also, if the whiskers are very long, then the data possesses outliers.
When data is analyzed in a box plot or chart, it can then be used to make conclusions on the experiment. These conclusions will either support or refute a hypothesis. It is important to note that hypothesis cannot be proven support can only be added for them or against them. Even scientific theories such as gravity are not proven: they are just supported by a wealth of experiments and knowledge.
This brings us to the basis of this question. We need to make a conclusion based on the results of a simulation. Initially, we can see that the treatments had some effect due to the differences between the abscisic acid plot and the control plot; therefore, any choice that said there was not an effect can be disregarded. In the graph, we can clearly see that abscisic acid inhibits plant growth compared to the control; therefore, we can say, "abscisic acid inhibits plant growth." When we compare the control group with the treatment group that received both gibberellins and abscisic acid, we can see that they are comparable. This means that we can infer the following: "gibberellins can counteract abscisic acid inhibition." The correct choice is: "Yes, abscisic acid inhibits plant growth and gibberellins can counteract growth inhibition."
Example Question #1 : Evaluate Reports Based On Data: Ccss.Math.Content.Hss Ic.B.6
A high school decides to use a standardized test to evaluate the general knowledge of all of its students. Each grade contains 100 students and every grade takes the same exam. The scores were evaluated and the following box plots for each grade were created:
Based on this report, we can infer which of the following regarding the junior class?
The test scores are bottom skewed
The test scores are top skewed
None of these
The test scores are uniform
The test scores are symmetrical
The test scores are bottom skewed
We know that the scientific method starts with an observation of a phenomenon. Next, hypotheses are developed and tested using simulations and experiments. Once data are collected they can be used to make conclusions through statistical analysis. In this question, we are asked to analyze the distribution of comparative box plots.
First, let's look at how box plots are used to analyze data.
Box plots are broken into five main parts that create four primary regions. Each region contains a quarter of the data in the plot. The plot is initially broken into two regions using the following values: the minimum value, the maximum value, and the median or average of the set. Next, the minimum and median values are divided by the median of the lower half of the data or the first/lower quartile. Likewise, the median and the maximum values are divided by the median of the upper half of the data set or third/upper quartile. If the box is bigger, then there is a greater variance or spread in the data. Also, if the whiskers are very long, then the data possesses outliers.
Next, let's observe how data are distributed in box plots.
A uniform distribution indicates that all values are the same. A symmetrical distribution means that each box in the plot encompasses the same area; furthermore, each whisker (i.e. the line between Q3 and the Maximum as well as the line between Q1 and the Minimum) are the same length. This means that the data is evenly distributed and follows a normal or bell curve distribution. Box plots can also be skewed: either top or bottom skewed. When a plot is bottom or negatively skewed, the distance from the median to the minimum is greater than the distance from the median to the maximum. Even though each box contains the same amount of the data (i.e. twenty-five percent), the box closer to the bottom covers more area than the one closer to the top of the chart. In other words the data is being pulled to the negative end of the chart by outlying data points, while the positive points are clustered closer together at the top of the chart. On the other hand, a plot is top or positively skewed when the distance from the median to the maximum is greater than the distance from the median to the minimum. Even though each box contains the same amount of the data (i.e. twenty-five percent), the box closer to the top covers more area than the one closer to the bottom of the chart. In other words the data is being pulled to the positive end of the chart by outlying data points, while the negative points are clustered closer together at the bottom of the chart.
When data is analyzed in a box plot or chart, it can then be used to make conclusions on the experiment. These conclusions will either support or refute a hypothesis. It is important to note that hypothesis cannot be proven only support can be added for them or against them. Even scientific theories such as gravity are not proven: they are just supported by a wealth of experiments and knowledge.
Now, let's answer the question. When we look at the box plot for the juniors we can see that the distance from the median to the minimum is greater than the distance from the median to the maximum: therefore, the plot is bottom or negatively skewed.
Example Question #217 : High School: Statistics & Probability
A high school decides to use a standardized test to evaluate the general knowledge of all of its students. Each grade contains 100 students and every grade takes the same exam. The scores were evaluated and the following box plots for each grade were created:
Based on this report we can infer which of the following regarding the junior class?
The test scores are symmetrical
The test scores are bottom skewed
None of these
The test scores are top skewed
The test scores are uniform
The test scores are bottom skewed
We know that the scientific method starts with the observation of a phenomenon. Next, hypotheses are developed and tested using simulations and experiments. Once data are collected they can be used to make conclusions through statistical analysis. In this question, we are asked to analyze the distribution of comparative box plots.
First, let's look at how box plots are used to analyze data.
Box plots are broken into five main parts that create four primary region. Each region contains a quarter of the data in the plot. The plot is broken into three initial regions: the minimum value, the maximum value, and the median or average of the set. Next the minimum and median are divided by the median of the lower half of the data or the first/lower quartile. Likewise the median and the maximum are divided by the median of the upper half of the data set or third/upper quartile. If the box is bigger, then there is a greater variance or spread in the data. Also, if the whiskers are very long, then the data possesses outliers.
Next, let's observe how data are distributed in box plots.
A uniform distribution indicates that all values are the same. A symmetrical distribution means that each box in the plot encompasses the same area; furthermore, each whisker (i.e. the line between Q3 and the Maximum as well as the line between Q1 and the Minimum) are the same length. This means that the data is evenly distributed and follows a normal or bell curve distribution. Box plots can also be skewed: either top or bottom skewed. When a plot is bottom or negatively skewed, the distance from the median to the minimum is greater than the distance from the median to the maximum. Even though each box contains the same amount of the data (i.e. twenty-five percent), the box closer to the bottom covers more area than the one closer to the top of the chart. In other words the data is being pulled to the negative end of the chart by outlying data points, while the positive points are clustered closer together at the top of the chart. On the other hand, a plot is top or positively skewed when the distance from the median to the maximum is greater than the distance from the median to the minimum. Even though each box contains the same amount of the data (i.e. twenty-five percent), the box closer to the top covers more area than the one closer to the bottom of the chart. In other words the data is being pulled to the positive end of the chart by outlying data points, while the negative points are clustered closer together at the bottom of the chart.
When data is analyzed in a box plot or chart, it can then be used to make conclusions on the experiment. These conclusions will either support or refute a hypothesis. It is important to note that hypothesis cannot be proven only support can be added for them or against them. Even scientific theories such as gravity are not proven: they are just supported by a wealth of experiments and knowledge.
Now, let's answer the question. When we look at the box plot for the juniors we can see that the distance from the median to the minimum is greater than the distance from the median to the maximum: therefore, the plot is bottom or negatively skewed.
Example Question #31 : Making Inferences & Justifying Conclusions
A high school decides to use a standardized test to evaluate the general knowledge of all of its students. Each grade contains 100 students and every grade takes the same exam. The scores were evaluated and the following box plots for each grade were created:
Based on this report, we can infer which of the following regarding the sophomore class?
The test scores are bottom skewed
None of these
The test scores are top skewed
The test scores are uniform
The test scores are symmetrical
The test scores are bottom skewed
We know that the scientific method starts with an observation of a phenomenon. Next, hypotheses are developed and tested using simulations and experiments. Once data are collected they can be used to make conclusions through statistical analysis. In this question, we are asked to analyze the distribution of comparative box plots.
First, let's look at how box plots are used to analyze data.
Box plots are broken into five main parts that create four primary regions. Each region contains a quarter of the data in the plot. The plot is initially broken into two regions using the following values: the minimum value, the maximum value, and the median or average of the set. Next, the minimum and median values are divided by the median of the lower half of the data or the first/lower quartile. Likewise, the median and the maximum values are divided by the median of the upper half of the data set or third/upper quartile. If the box is bigger, then there is a greater variance or spread in the data. Also, if the whiskers are very long, then the data possesses outliers.
Next, let's observe how data are distributed in box plots.
A uniform distribution indicates that all values are the same. A symmetrical distribution means that each box in the plot encompasses the same area; furthermore, each whisker (i.e. the line between Q3 and the Maximum as well as the line between Q1 and the Minimum) are the same length. This means that the data is evenly distributed and follows a normal or bell curve distribution. Box plots can also be skewed: either top or bottom skewed. When a plot is bottom or negatively skewed, the distance from the median to the minimum is greater than the distance from the median to the maximum. Even though each box contains the same amount of the data (i.e. twenty-five percent), the box closer to the bottom covers more area than the one closer to the top of the chart. In other words the data is being pulled to the negative end of the chart by outlying data points, while the positive points are clustered closer together at the top of the chart. On the other hand, a plot is top or positively skewed when the distance from the median to the maximum is greater than the distance from the median to the minimum. Even though each box contains the same amount of the data (i.e. twenty-five percent), the box closer to the top covers more area than the one closer to the bottom of the chart. In other words the data is being pulled to the positive end of the chart by outlying data points, while the negative points are clustered closer together at the bottom of the chart.
When data is analyzed in a box plot or chart, it can then be used to make conclusions on the experiment. These conclusions will either support or refute a hypothesis. It is important to note that hypothesis cannot be proven only support can be added for them or against them. Even scientific theories such as gravity are not proven: they are just supported by a wealth of experiments and knowledge.
Now, let's answer the question. When we look at the box plot for the sophomores we can see that the distance from the median to the minimum is greater than the distance from the median to the maximum: therefore, the plot is bottom or negatively skewed.
Example Question #219 : High School: Statistics & Probability
A high school decides to use a standardized test to evaluate the general knowledge of all of its students. Each grade contains 100 students and every grade takes the same exam. The scores were evaluated and the following box plots for each grade were created:
Based on this report, we can infer which of the following regarding the senior class?
The test scores are symmetrical
None of these
The test scores are top skewed
The test scores are uniform
The test scores are bottom skewed
The test scores are top skewed
We know that the scientific method starts with an observation of a phenomenon. Next, hypotheses are developed and tested using simulations and experiments. Once data are collected they can be used to make conclusions through statistical analysis. In this question, we are asked to analyze the distribution of comparative box plots.
First, let's look at how box plots are used to analyze data.
Box plots are broken into five main parts that create four primary regions. Each region contains a quarter of the data in the plot. The plot is initially broken into two regions using the following values: the minimum value, the maximum value, and the median or average of the set. Next, the minimum and median values are divided by the median of the lower half of the data or the first/lower quartile. Likewise, the median and the maximum values are divided by the median of the upper half of the data set or third/upper quartile. If the box is bigger, then there is a greater variance or spread in the data. Also, if the whiskers are very long, then the data possesses outliers.
Next, let's observe how data are distributed in box plots.
A uniform distribution indicates that all values are the same. A symmetrical distribution means that each box in the plot encompasses the same area; furthermore, each whisker (i.e. the line between Q3 and the Maximum as well as the line between Q1 and the Minimum) are the same length. This means that the data is evenly distributed and follows a normal or bell curve distribution. Box plots can also be skewed: either top or bottom skewed. When a plot is bottom or negatively skewed, the distance from the median to the minimum is greater than the distance from the median to the maximum. Even though each box contains the same amount of the data (i.e. twenty-five percent), the box closer to the bottom covers more area than the one closer to the top of the chart. In other words the data is being pulled to the negative end of the chart by outlying data points, while the positive points are clustered closer together at the top of the chart. On the other hand, a plot is top or positively skewed when the distance from the median to the maximum is greater than the distance from the median to the minimum. Even though each box contains the same amount of the data (i.e. twenty-five percent), the box closer to the top covers more area than the one closer to the bottom of the chart. In other words the data is being pulled to the positive end of the chart by outlying data points, while the negative points are clustered closer together at the bottom of the chart.
When data is analyzed in a box plot or chart, it can then be used to make conclusions on the experiment. These conclusions will either support or refute a hypothesis. It is important to note that hypothesis cannot be proven only support can be added for them or against them. Even scientific theories such as gravity are not proven: they are just supported by a wealth of experiments and knowledge.
Now, let's answer the question. When we look at the box plot for the seniors we can see that the distance from the median to the minimum is less than the distance from the median to the maximum: therefore, the plot is top or positively skewed.
Example Question #32 : Making Inferences & Justifying Conclusions
A high school decides to use a standardized test to evaluate the general knowledge of all of its students. Each grade contains 100 students and every grade takes the same exam. The scores were evaluated and the following box plots for each grade were created:
Based on this report, we can infer which of the following regarding the sophomore class?
The test scores are top skewed
None of these
The test scores are uniform
The test scores are symmetrical
The test scores are bottom skewed
The test scores are top skewed
We know that the scientific method starts with an observation of a phenomenon. Next, hypotheses are developed and tested using simulations and experiments. Once data are collected they can be used to make conclusions through statistical analysis. In this question, we are asked to analyze the distribution of comparative box plots.
First, let's look at how box plots are used to analyze data.
Box plots are broken into five main parts that create four primary regions. Each region contains a quarter of the data in the plot. The plot is initially broken into two regions using the following values: the minimum value, the maximum value, and the median or average of the set. Next, the minimum and median values are divided by the median of the lower half of the data or the first/lower quartile. Likewise, the median and the maximum values are divided by the median of the upper half of the data set or third/upper quartile. If the box is bigger, then there is a greater variance or spread in the data. Also, if the whiskers are very long, then the data possesses outliers.
Next, let's observe how data are distributed in box plots.
A uniform distribution indicates that all values are the same. A symmetrical distribution means that each box in the plot encompasses the same area; furthermore, each whisker (i.e. the line between Q3 and the Maximum as well as the line between Q1 and the Minimum) are the same length. This means that the data is evenly distributed and follows a normal or bell curve distribution. Box plots can also be skewed: either top or bottom skewed. When a plot is bottom or negatively skewed, the distance from the median to the minimum is greater than the distance from the median to the maximum. Even though each box contains the same amount of the data (i.e. twenty-five percent), the box closer to the bottom covers more area than the one closer to the top of the chart. In other words the data is being pulled to the negative end of the chart by outlying data points, while the positive points are clustered closer together at the top of the chart. On the other hand, a plot is top or positively skewed when the distance from the median to the maximum is greater than the distance from the median to the minimum. Even though each box contains the same amount of the data (i.e. twenty-five percent), the box closer to the top covers more area than the one closer to the bottom of the chart. In other words the data is being pulled to the positive end of the chart by outlying data points, while the negative points are clustered closer together at the bottom of the chart.
When data is analyzed in a box plot or chart, it can then be used to make conclusions on the experiment. These conclusions will either support or refute a hypothesis. It is important to note that hypothesis cannot be proven only support can be added for them or against them. Even scientific theories such as gravity are not proven: they are just supported by a wealth of experiments and knowledge.
Now, let's answer the question. When we look at the box plot for the sophomores we can see that the distance from the median to the minimum is less than the distance from the median to the maximum: therefore, the plot is top or positively skewed.
All Common Core: High School - Statistics and Probability Resources
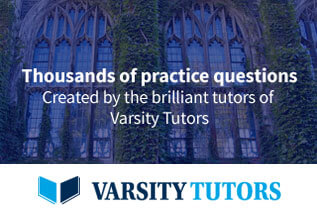