All Common Core: High School - Statistics and Probability Resources
Example Questions
Example Question #101 : Interpreting Categorical & Quantitative Data
A social scientist measures the correlation between an individual's socio-economic status and highest level of education obtained. The scientists plots these points and creates the following graph.
Which of the following best describes how the variables in the graph are related to one another?
Weak or moderately positive
Strong positive
Weak or moderately negative
Strong negative
No relationship
Weak or moderately negative
In order to solve this problem, we need to understand the common associations between variables with linear relationships. Scatter plots are used to plot data points with two variables. A correlation exists when there is an observable relationship between points. There are several common relationships between correlations. They include the following: weak positive, strong positive, weak negative, strong negative, and random relationships.
Let's first look at whether a relationship is positive or negative. Positive relationships flow upward vertically from the left to the right of the horizontal axis, while negative relationships flow downward. Next, let's discuss how to tell if a relationship is strong or weak/moderate. A strong relationship exists when the plotted points are clustered close together to one another; whereas, a weak relationship is characterized by a greater amount of spread between points. Last, a random relationship occurs when there is no discernible pattern of association between points.
Before we solve this question, let's look at each of these relationships in turn.
Weak or moderately positive
A weak or moderately positive relationship exists when points increase from the left to the right but possess a large spread between points away from the trend line.
Strong positive
A strong positive relationship exists when points increase from the left to the right and are clustered tightly around the trend line.
Weak or moderately negative
A weak or moderately negative relationship exists when points decrease from the left to the right but possess a large spread between points away from the trend line.
Strong negative
A strong negative relationship exists when points decrease from the left to the right and are clustered tightly around the trend line.
No or random relationship
A random relationship or spread exists when the data does not increase or decrease from the left to the right; furthermore, a nearly horizontal trend line characterizes these graphs. They possess a great spread and appear random in nature of associations between points.
Now, let's focus on our question. The question states that several variables were plotted against one another and the following graph was produced:
We can see that the trend line decreases from the left to the right; therefore, there is a negative relationship between points. Also, we can see that the points are not closely clustered together. This graph depicts a weak or moderately negative relationship.
Example Question #102 : Interpreting Categorical & Quantitative Data
A social scientist measures the correlation between an individual's socio-economic status and highest level of education obtained. The scientists plots these points and creates the following graph.
Which of the following best describes how the variables in the graph are related to one another?
Weak or moderately negative
Strong positive
Strong negative
No relationship
Weak or moderately positive
Weak or moderately negative
In order to solve this problem, we need to understand the common associations between variables with linear relationships. Scatter plots are used to plot data points with two variables. A correlation exists when there is an observable relationship between points. There are several common relationships between correlations. They include the following: weak positive, strong positive, weak negative, strong negative, and random relationships.
Let's first look at whether a relationship is positive or negative. Positive relationships flow upward vertically from the left to the right of the horizontal axis, while negative relationships flow downward. Next, let's discuss how to tell if a relationship is strong or weak/moderate. A strong relationship exists when the plotted points are clustered close together to one another; whereas, a weak relationship is characterized by a greater amount of spread between points. Last, a random relationship occurs when there is no discernible pattern of association between points.
Before we solve this question, let's look at each of these relationships in turn.
Weak or moderately positive
A weak or moderately positive relationship exists when points increase from the left to the right but possess a large spread between points away from the trend line.
Strong positive
A strong positive relationship exists when points increase from the left to the right and are clustered tightly around the trend line.
Weak or moderately negative
A weak or moderately negative relationship exists when points decrease from the left to the right but possess a large spread between points away from the trend line.
Strong negative
A strong negative relationship exists when points decrease from the left to the right and are clustered tightly around the trend line.
No or random relationship
A random relationship or spread exists when the data does not increase or decrease from the left to the right; furthermore, a nearly horizontal trend line characterizes these graphs. They possess a great spread and appear random in nature of associations between points.
Now, let's focus on our question. The question states that several variables were plotted against one another and the following graph was produced:
We can see that the trend line decreases from the left to the right; therefore, there is a negative relationship between points. Also, we can see that the points are not closely clustered together. This graph depicts a weak or moderately negative relationship.
Example Question #103 : Interpreting Categorical & Quantitative Data
A social scientist measures the correlation between an individual's socio-economic status and highest level of education obtained. The scientists plots these points and creates the following graph.
Which of the following best describes how the variables in the graph are related to one another?
No relationship
Strong positive
Weak or moderately negative
Strong negative
Weak or moderately positive
Weak or moderately positive
In order to solve this problem, we need to understand the common associations between variables with linear relationships. Scatter plots are used to plot data points with two variables. A correlation exists when there is an observable relationship between points. There are several common relationships between correlations. They include the following: weak positive, strong positive, weak negative, strong negative, and random relationships.
Let's first look at whether a relationship is positive or negative. Positive relationships flow upward vertically from the left to the right of the horizontal axis, while negative relationships flow downward. Next, let's discuss how to tell if a relationship is strong or weak/moderate. A strong relationship exists when the plotted points are clustered close together to one another; whereas, a weak relationship is characterized by a greater amount of spread between points. Last, a random relationship occurs when there is no discernible pattern of association between points.
Before we solve this question, let's look at each of these relationships in turn.
Weak or moderately positive
A weak or moderately positive relationship exists when points increase from the left to the right but possess a large spread between points away from the trend line.
Strong positive
A strong positive relationship exists when points increase from the left to the right and are clustered tightly around the trend line.
Weak or moderately negative
A weak or moderately negative relationship exists when points decrease from the left to the right but possess a large spread between points away from the trend line.
Strong negative
A strong negative relationship exists when points decrease from the left to the right and are clustered tightly around the trend line.
No or random relationship
A random relationship or spread exists when the data does not increase or decrease from the left to the right; furthermore, a nearly horizontal trend line characterizes these graphs. They possess a great spread and appear random in nature of associations between points.
Now, let's focus on our question. The question states that several variables were plotted against one another and the following graph was produced:
We can see that the trend line increases from the left to the right; therefore, there is a positive relationship between points. Also, we can see that the points are not closely clustered together. This graph depicts a weak or moderately positive relationship.
Example Question #104 : Interpreting Categorical & Quantitative Data
A social scientist measures the correlation between an individual's socio-economic status and highest level of education obtained. The scientists plots these points and creates the following graph.
Which of the following best describes how the variables in the graph are related to one another?
Strong negative
No relationship
Weak or moderately positive
Strong positive
Weak or moderately negative
No relationship
In order to solve this problem, we need to understand the common associations between variables with linear relationships. Scatter plots are used to plot data points with two variables. A correlation exists when there is an observable relationship between points. There are several common relationships between correlations. They include the following: weak positive, strong positive, weak negative, strong negative, and random relationships.
Let's first look at whether a relationship is positive or negative. Positive relationships flow upward vertically from the left to the right of the horizontal axis, while negative relationships flow downward. Next, let's discuss how to tell if a relationship is strong or weak/moderate. A strong relationship exists when the plotted points are clustered close together to one another; whereas, a weak relationship is characterized by a greater amount of spread between points. Last, a random relationship occurs when there is no discernible pattern of association between points.
Before we solve this question, let's look at each of these relationships in turn.
Weak or moderately positive
A weak or moderately positive relationship exists when points increase from the left to the right but possess a large spread between points away from the trend line.
Strong positive
A strong positive relationship exists when points increase from the left to the right and are clustered tightly around the trend line.
Weak or moderately negative
A weak or moderately negative relationship exists when points decrease from the left to the right but possess a large spread between points away from the trend line.
Strong negative
A strong negative relationship exists when points decrease from the left to the right and are clustered tightly around the trend line.
No or random relationship
A random relationship or spread exists when the data does not increase or decrease from the left to the right; furthermore, a nearly horizontal trend line characterizes these graphs. They possess a great spread and appear random in nature of associations between points.
Now, let's focus on our question. The question states that several variables were plotted against one another and the following graph was produced:
We can see that the trend line is horizontal from the left to the right; therefore, there is no relationship between points. Also, we can see that the points are not closely clustered together. This graph depicts no relationship.
Example Question #105 : High School: Statistics & Probability
Researchers study a group of thirty males. They collect data on their weight and the length of time it takes them to run one mile. The data was recorded in the following table:
Afterwards, the researchers plotted the point on a scatter plot and fit a trend line with an equation to the data.
Based on this data, estimate how long it will take a male that weighs 185 pounds to run a mile.
When data are presented using a scatter plot that is fitted with a trend line, we can calculate estimations based on the association between variables. Several conditions must be met before one can use a scatter plot to make estimates off of correlations.
First, the points must possess some type of relationship between one another. This relationship can be positive or negative. Positive relationships occur when data move upwards from the left side to the right side of the graph; however, when the data slopes downward the relationship is negative. Second, we must identify if the relationship is strong or weak. A strong correlation exists when the data is clustered closer together and the trend line. On the other hand, a weak correlation occurs when data is spread apart from each other and the trend line. After we have evaluated these characteristics of the graph, we can use the scatter plot to make predictions.
How do we make predictions? Predictions are made using several methods: qualitative and quantitative observations. One can qualitatively use the associations present in the graph to make estimates based on spread, clustering, and the trend line’s position. Spread between points in a given area can provide a range of values for a given coordinate, while clustering of plots in a given area can give an average value for a given coordinate. On the other hand, the trend line can be used to estimate points by drawing lines that intersect from each axis. Last, we can quantitatively estimate a point using the equation of the trend line and solving for either the x or y variable.
Let’s work with these methods and solve the question.
First let’s observe if we can make a prediction using the following data:
First, let’s observe if we can make a prediction using the following data:
We can see that the data possesses a positive correlation: that is, as the runner’s weight increases then so does the time it takes them to run a mile. Also, we can see that the points are weakly to moderately clustered with one another and the trend line; therefore, we can use qualitative and quantitative means to estimate how fast a 185 pound male can run a mile.
Next, let’s make a qualitative estimate by drawing a line from 185 pounds on the x-axis to the trend line. Next, we will draw a line from that point to the y-axis. This will qualitatively estimate our value. Observe this method below:
From this information, we can make the prediction that a male of this weight should be able to run a mile in just under ten minutes.
Now, lets predict this value quantitatively using the equation of the line:
Plug in 185 for the x-coordinate.
Solve.
Round to two decimal places.
According to the data, we can predict that it would take a male 9.71 minutes for a 185 pound male to run a single mile.
Remember, we can also use this method to estimate the y-value of the data.
Example Question #1 : Fit A Function To The Data: Ccss.Math.Content.Hss Id.B.6a
Researchers study a group of thirty males. They collect data on their weight and the length of time it takes them to run one mile. The data was recorded in the following table:
Afterwards, the researchers plotted the data on a scatter plot and fit a trend line with an equation for the data.
Based on this data, estimate how long it will take a male that weighs 185 pounds to run a mile?
When data are presented using a scatter plot that is fitted with a trend line, we can calculate estimations based on the association between variables. Several conditions must be met before one can use a scatter plot to make estimates off of correlations.
First, the points must possess some type of relationship between one another. This relationship can be positive or negative. Positive relationships occur when data move upwards from the left side to the right side of the graph; however, when the data slopes downward the relationship is negative. Second, we must identify if the relationship is strong or weak. A strong correlation exists when the data is clustered closer together and the trend line. On the other hand, a weak correlation occurs when data is spread apart from each other and the trend line. After we have evaluated these characteristics of the graph, we can use the scatter plot to make predictions.
How do we make predictions? Predictions are made using several methods: qualitative and quantitative observations. One can qualitatively use the associations present in the graph to make estimates based on spread, clustering, and the trend line’s position. Spread between points in a given area can provide a range of values for a given coordinate, while clustering of plots in a given area can give an average value for a given coordinate. On the other hand, the trend line can be used to estimate points by drawing lines that intersect from each axis. Last, we can quantitatively estimate a point using the equation of the trend line and solving for either the x- or y-variable.
Let’s work with these methods and solve the question.
First let’s observe if we can make a prediction using the following data:
We can see that the data possesses a positive correlation: that is, as the runner’s weight increases then so does the time it takes them to run a mile. Also, we can see that the points are weakly to moderately clustered with one another and the trend line; therefore, we can use qualitative and quantitative means to estimate how fast a 185 pound male can run a mile.
Next, let’s make a qualitative estimate by drawing a line from 185 pounds on the x-axis to the trend line. Next, we will draw a line from that point to the y-axis. This will qualitatively estimate our value. Observe this method below:
From this information, we can make the prediction that a male of this weight should be able to run a mile in just under ten minutes.
Now, lets predict this value quantitatively using the equation of the line:
Plug in 185 for the x-coordinate.
Solve.
Round to two decimal places.
According to the data, we can predict that it would take a male 9.71 minutes for a 185 pound male to run a single mile.
Remember, we can also use this method to estimate the y-value of the data.
Example Question #1 : Fit A Function To The Data: Ccss.Math.Content.Hss Id.B.6a
Researchers study a group of thirty males. They collect data on their weight and the length of time it takes them to run one mile. The data was recorded in the following table:
Afterwards, the researchers plotted the data on a scatter plot and fit a trend line with an equation for the data.
Based on this data, estimate how long it will take a male that weighs 185 pounds to run a mile?
When data are presented using a scatter plot that is fitted with a trend line, we can calculate estimations based on the association between variables. Several conditions must be met before one can use a scatter plot to make estimates off of correlations.
First, the points must possess some type of relationship between one another. This relationship can be positive or negative. Positive relationships occur when data move upwards from the left side to the right side of the graph; however, when the data slopes downward the relationship is negative. Second, we must identify if the relationship is strong or weak. A strong correlation exists when the data is clustered closer together and the trend line. On the other hand, a weak correlation occurs when data is spread apart from each other and the trend line. After we have evaluated these characteristics of the graph, we can use the scatter plot to make predictions.
How do we make predictions? Predictions are made using several methods: qualitative and quantitative observations. One can qualitatively use the associations present in the graph to make estimates based on spread, clustering, and the trend line’s position. Spread between points in a given area can provide a range of values for a given coordinate, while clustering of plots in a given area can give an average value for a given coordinate. On the other hand, the trend line can be used to estimate points by drawing lines that intersect from each axis. Last, we can quantitatively estimate a point using the equation of the trend line and solving for either the x- or y-variable.
Let’s work with these methods and solve the question.
First let’s observe if we can make a prediction using the following data:
We can see that the data possesses a positive correlation: that is, as the runner’s weight increases then so does the time it takes them to run a mile. Also, we can see that the points are weakly to moderately clustered with one another and the trend line; therefore, we can use qualitative and quantitative means to estimate how fast a 185 pound male can run a mile.
Next, let’s make a qualitative estimate by drawing a line from 185 pounds on the x-axis to the trend line. Next, we will draw a line from that point to the y-axis. This will qualitatively estimate our value. Observe this method below:
From this information, we can make the prediction that a male of this weight should be able to run a mile in just under ten minutes.
Now, lets predict this value quantitatively using the equation of the line:
Plug in 185 for the x-coordinate.
Solve.
Round to two decimal places.
According to the data, we can predict that it would take a male 9.71 minutes for a 185 pound male to run a single mile.
Remember, we can also use this method to estimate the y-value of the data.
Example Question #1 : Fit A Function To The Data: Ccss.Math.Content.Hss Id.B.6a
Researchers study a group of thirty males. They collect data on their weight and the length of time it takes them to run one mile. The data was recorded in the following table:
Afterwards, the researchers plotted the data on a scatter plot and fit a trend line with an equation for the data.
If the best fit line is estimate how long it will take a male that weighs
pounds to run a mile?
When data are presented using a scatter plot that is fitted with a trend line, we can calculate estimations based on the association between variables. Several conditions must be met before one can use a scatter plot to make estimates off of correlations.
First, the points must possess some type of relationship between one another. This relationship can be positive or negative. Positive relationships occur when data move upwards from the left side to the right side of the graph; however, when the data slopes downward the relationship is negative. Second, we must identify if the relationship is strong or weak. A strong correlation exists when the data is clustered closer together and the trend line. On the other hand, a weak correlation occurs when data is spread apart from each other and the trend line. After we have evaluated these characteristics of the graph, we can use the scatter plot to make predictions.
How do we make predictions? Predictions are made using several methods: qualitative and quantitative observations. One can qualitatively use the associations present in the graph to make estimates based on spread, clustering, and the trend line’s position. Spread between points in a given area can provide a range of values for a given coordinate, while clustering of plots in a given area can give an average value for a given coordinate. On the other hand, the trend line can be used to estimate points by drawing lines that intersect from each axis. Last, we can quantitatively estimate a point using the equation of the trend line and solving for either the x- or y-variable.
Let’s work with these methods and solve the question.
First let’s observe if we can make a prediction using the following data:
We can see that the data possesses a positive correlation: that is, as the runner’s weight increases then so does the time it takes them to run a mile. Also, we can see that the points are weakly to moderately clustered with one another and the trend line; therefore, we can use qualitative and quantitative means to estimate how fast a 125 pound male can run a mile.
Next, let’s make a qualitative estimate by drawing a line from 125 pounds on the x-axis to the trend line. Next, we will draw a line from that point to the y-axis. This will qualitatively estimate our value. Observe this method below:
Now, lets predict this value quantitatively using the equation of the line:
Plug in 125 for the x-coordinate.
Solve
Round to two decimal places.
According to the data, we can predict that it would take a male 9.94 minutes for a 125 pound male to run a single mile.
Remember, we can also use this method to estimate the y-value of the data.
Example Question #2 : Fit A Function To The Data: Ccss.Math.Content.Hss Id.B.6a
Researchers study a group of thirty males. They collect data on their weight and the length of time it takes them to run one mile. The data was recorded in the following table:
Afterwards, the researchers plotted the data on a scatter plot and fit a trend line with an equation for the data.
If the best fit line is estimate how long it will take a male that weighs
pounds to run a mile?
When data are presented using a scatter plot that is fitted with a trend line, we can calculate estimations based on the association between variables. Several conditions must be met before one can use a scatter plot to make estimates off of correlations.
First, the points must possess some type of relationship between one another. This relationship can be positive or negative. Positive relationships occur when data move upwards from the left side to the right side of the graph; however, when the data slopes downward the relationship is negative. Second, we must identify if the relationship is strong or weak. A strong correlation exists when the data is clustered closer together and the trend line. On the other hand, a weak correlation occurs when data is spread apart from each other and the trend line. After we have evaluated these characteristics of the graph, we can use the scatter plot to make predictions.
How do we make predictions? Predictions are made using several methods: qualitative and quantitative observations. One can qualitatively use the associations present in the graph to make estimates based on spread, clustering, and the trend line’s position. Spread between points in a given area can provide a range of values for a given coordinate, while clustering of plots in a given area can give an average value for a given coordinate. On the other hand, the trend line can be used to estimate points by drawing lines that intersect from each axis. Last, we can quantitatively estimate a point using the equation of the trend line and solving for either the x- or y-variable.
Let’s work with these methods and solve the question.
First let’s observe if we can make a prediction using the following data:
We can see that the data possesses a positive correlation: that is, as the runner’s weight increases then so does the time it takes them to run a mile. Also, we can see that the points are weakly to moderately clustered with one another and the trend line; therefore, we can use qualitative and quantitative means to estimate how fast a 205 pound male can run a mile.
Next, let’s make a qualitative estimate by drawing a line from 205 pounds on the x-axis to the trend line. Next, we will draw a line from that point to the y-axis. This will qualitatively estimate our value. Observe this method below:
Now, lets predict this value quantitatively using the equation of the line:
Plug in 205 for the x-coordinate.
Solve
Round to two decimal places.
According to the data, we can predict that it would take a male 8.61 minutes for a 205 pound male to run a single mile.
Remember, we can also use this method to estimate the y-value of the data.
Example Question #3 : Fit A Function To The Data: Ccss.Math.Content.Hss Id.B.6a
Researchers study a group of thirty males. They collect data on their weight and the length of time it takes them to run one mile. The data was recorded in the following table:
Afterwards, the researchers plotted the data on a scatter plot and fit a trend line with an equation for the data.
If the best fit line is estimate how long it will take a male that weighs
pounds to run a mile?
When data are presented using a scatter plot that is fitted with a trend line, we can calculate estimations based on the association between variables. Several conditions must be met before one can use a scatter plot to make estimates off of correlations.
First, the points must possess some type of relationship between one another. This relationship can be positive or negative. Positive relationships occur when data move upwards from the left side to the right side of the graph; however, when the data slopes downward the relationship is negative. Second, we must identify if the relationship is strong or weak. A strong correlation exists when the data is clustered closer together and the trend line. On the other hand, a weak correlation occurs when data is spread apart from each other and the trend line. After we have evaluated these characteristics of the graph, we can use the scatter plot to make predictions.
How do we make predictions? Predictions are made using several methods: qualitative and quantitative observations. One can qualitatively use the associations present in the graph to make estimates based on spread, clustering, and the trend line’s position. Spread between points in a given area can provide a range of values for a given coordinate, while clustering of plots in a given area can give an average value for a given coordinate. On the other hand, the trend line can be used to estimate points by drawing lines that intersect from each axis. Last, we can quantitatively estimate a point using the equation of the trend line and solving for either the x- or y-variable.
Let’s work with these methods and solve the question.
First let’s observe if we can make a prediction using the following data:
We can see that the data possesses a positive correlation: that is, as the runner’s weight increases then so does the time it takes them to run a mile. Also, we can see that the points are weakly to moderately clustered with one another and the trend line; therefore, we can use qualitative and quantitative means to estimate how fast a 188 pound male can run a mile.
Next, let’s make a qualitative estimate by drawing a line from 188 pounds on the x-axis to the trend line. Next, we will draw a line from that point to the y-axis. This will qualitatively estimate our value. Observe this method below:
Now, lets predict this value quantitatively using the equation of the line:
Plug in 188 for the x-coordinate.
Solve
Round to two decimal places.
According to the data, we can predict that it would take a male 8.79 minutes for a 188 pound male to run a single mile.
Remember, we can also use this method to estimate the y-value of the data.
All Common Core: High School - Statistics and Probability Resources
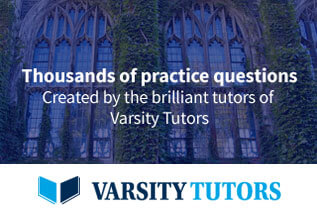