All Common Core: High School - Statistics and Probability Resources
Example Questions
Example Question #1 : Use Probabilities To Make Fair Decisions: Ccss.Math.Content.Hss Md.B.6
Lauren has a computer programming assignment to create a random number generator that generates numbers on the interval .
Which of the following statements must be TRUE about the random number generator if it is fair?
Each number between 0 and 1 is equally likely to occur.
There should be more numbers generated around 0.5 than around 0 or 1, because 0.5 is the median.
Each number between 0 and 1 must have a different probability of occuring.
The numbers 0 and 1 will not occur.
There should be more numbers generated around 0.5 than around 0 or 1, because 0.5 is the mean.
Each number between 0 and 1 is equally likely to occur.
Example Question #1 : Use Probabilities To Make Fair Decisions: Ccss.Math.Content.Hss Md.B.6
Referees at a professional football game need to choose a coin for the pre-game coin toss. Studies have shown that a fair coin possesses the following standard deviation:
They test five coins by flipping them one hundred times and noting how many times each lands on heads or tails. Which of the following coin is least fair according to the standard deviation?
Coin 4
Coins 2 and 5
Coin 1
Coins 1 and 4
Coin 3
Coin 3
This standard relates to our ability to use probability to make fair decisions. Other standards used the expected means formula in order to calculate expected payoffs of games, lottery drawings, insurance policies, business ventures, and others. This information was then used in order to help us make decisions on whether to play games, invest in a company, or choose an insurance policy. Likewise, the essence of this standard is related to fairness or using probabilities to make fair decisions. In order to answer this question we need to discuss probabilities and the concept of fairness in events. First, we will discuss probabilities in a general sense.
A probability is generally defined as the chances or likelihood of an event occurring. It is calculated by identifying two components: the event and the sample space. The event is defined as the favorable outcome or success that we wish to observe. On the other hand, the sample space is defined as the set of all possible outcomes for the event. Mathematically we calculate probabilities by dividing the event by the sample space:
Let's use a simple example: the rolling of a die. We want to know the probability of rolling a one. We know that the sample space is six because there are six sides or outcomes to the die. Also, we know that there is only a single side with a value of one; therefore,
Now, let's convert this into a percentage:
Probabilities expressed in fraction form will have values between zero and one. One indicates that an event will definitely occur, while zero indicates that an event will not occur. Likewise, probabilities expressed as percentages possess values between zero and one hundred percent where probabilities closer to zero are unlikely to occur and those close to one hundred percent are more likely to occur.
According to this logic, we would expect to roll a particular number on a die one out of every six rolls; however, we may roll the same number multiple times or not at all in six rolls. This discrepancy creates a difference between the expected mean and the actual mean. The expected mean is a hypothetical calculation that assumes a very large sample size and no intervening variables (such as differing forces on the roll and changes in the friction of the surface the die is rolled upon between rolls). Under these conditions we can calculate that any number on the die in a "perfect world" should be one out of six. On the other hand the true or actual mean is calculated using "real" date. In these calculations we would roll a die a particular number of times and use it to develop a probability of rolling a particular number. It is important to note that, theoretically, actual means will eventually equal expected means over a large—or near infinite—amount of trials. In other words, over many many rolls we would eventually find that each number on the die has a one in six chance of being rolled.
Now, let's discuss how fairness is described in chance events. In this sense, fairness is associated with randomness. This means that there is no bias being imparted upon the decision in the event. In other words, there is no bias on the part of the individuals nor is there a bias on the part the device being used to produce the outcome of the event. This means that the decision produced from the chance event will be “fair.” It is important to note that deviations from expected probabilities may occur. For instance, a person may roll a six on a fair die six times in a row. According to probability, we would expect a die to land with the six-side facing upwards only once out of six rolls; however, the process of deviation can help explain this phenomenon.
Suppose we rolled a fair die ten times and recorded the distribution. Next, we decided to do this an infinite number of times. We would create a "bell" shaped curve. In this curve the center would represent events that are likely to occur, such as rolling each side of the die on average one out of six times. The tails or extremes of the curve represent unlikely events that may occur but are highly unlikely, such as rolling one side of the die six times in a row. The dead center of the curve represents the mean and the standard deviation measures how far—under standard conditions—we can expect a sample to deviate or vary from the mean. in other words, if a set of dice consistently roll the same value many times, then we can assume that they may have been rigged or altered.
Probability rests upon the concepts of fairness and randomness. These terms are not necessarily synonymous. Fairness relates to the devices being used (e.g. coin, deck of cards, or die). On the other hand, randomness is an essential component of any statistical measure and indicates that every outcome has an equal chance of occurring. Now, let's use this information to solve the problem.
In order to solve this problem, we need to calculate how the probability varies under standard conditions according to the standard deviation. We know that we have a one out of two chance of flipping a coin to heads or tails; therefore, we can write the following probabilities:
We know the standard deviation equals the following:
This means that the probability may vary by this amount; therefore, a fair coin may possess the following probabilities:
Only one coin possesses probabilities outside of this range: "coin 3." This coin posses the following probabilities:
Example Question #3 : Use Probabilities To Make Fair Decisions: Ccss.Math.Content.Hss Md.B.6
Referees at a professional football game need to choose a coin for the pre-game coin toss. Studies have shown that a fair coin possesses the following standard deviation:
They test five coins by flipping them one hundred times and noting how many times each lands on heads or tails. Which of the following coin is least fair according to the standard deviation?
Coins 1 and 4
Coin 1
Coin 4
Coins 2 and 5
Coin 3
Coin 3
This standard relates to our ability to use probability to make fair decisions. Other standards used the expected means formula in order to calculate expected payoffs of games, lottery drawings, insurance policies, business ventures, and others. This information was then used in order to help us make decisions on whether to play games, invest in a company, or choose an insurance policy. Likewise, the essence of this standard is related to fairness or using probabilities to make fair decisions. In order to answer this question we need to discuss probabilities and the concept of fairness in events. First, we will discuss probabilities in a general sense.
A probability is generally defined as the chances or likelihood of an event occurring. It is calculated by identifying two components: the event and the sample space. The event is defined as the favorable outcome or success that we wish to observe. On the other hand, the sample space is defined as the set of all possible outcomes for the event. Mathematically we calculate probabilities by dividing the event by the sample space:
Let's use a simple example: the rolling of a die. We want to know the probability of rolling a one. We know that the sample space is six because there are six sides or outcomes to the die. Also, we know that there is only a single side with a value of one; therefore,
Now, let's convert this into a percentage:
Probabilities expressed in fraction form will have values between zero and one. One indicates that an event will definitely occur, while zero indicates that an event will not occur. Likewise, probabilities expressed as percentages possess values between zero and one hundred percent where probabilities closer to zero are unlikely to occur and those close to one hundred percent are more likely to occur.
According to this logic, we would expect to roll a particular number on a die one out of every six rolls; however, we may roll the same number multiple times or not at all in six rolls. This discrepancy creates a difference between the expected mean and the actual mean. The expected mean is a hypothetical calculation that assumes a very large sample size and no intervening variables (such as differing forces on the roll and changes in the friction of the surface the die is rolled upon between rolls). Under these conditions we can calculate that any number on the die in a "perfect world" should be one out of six. On the other hand the true or actual mean is calculated using "real" date. In these calculations we would roll a die a particular number of times and use it to develop a probability of rolling a particular number. It is important to note that, theoretically, actual means will eventually equal expected means over a large—or near infinite—amount of trials. In other words, over many many rolls we would eventually find that each number on the die has a one in six chance of being rolled.
Now, let's discuss how fairness is described in chance events. In this sense, fairness is associated with randomness. This means that there is no bias being imparted upon the decision in the event. In other words, there is no bias on the part of the individuals nor is there a bias on the part the device being used to produce the outcome of the event. This means that the decision produced from the chance event will be “fair.” It is important to note that deviations from expected probabilities may occur. For instance, a person may roll a six on a fair die six times in a row. According to probability, we would expect a die to land with the six-side facing upwards only once out of six rolls; however, the process of deviation can help explain this phenomenon.
Suppose we rolled a fair die ten times and recorded the distribution. Next, we decided to do this an infinite number of times. We would create a "bell" shaped curve. In this curve the center would represent events that are likely to occur, such as rolling each side of the die on average one out of six times. The tails or extremes of the curve represent unlikely events that may occur but are highly unlikely, such as rolling one side of the die six times in a row. The dead center of the curve represents the mean and the standard deviation measures how far—under standard conditions—we can expect a sample to deviate or vary from the mean. in other words, if a set of dice consistently roll the same value many times, then we can assume that they may have been rigged or altered.
Probability rests upon the concepts of fairness and randomness. These terms are not necessarily synonymous. Fairness relates to the devices being used (e.g. coin, deck of cards, or die). On the other hand, randomness is an essential component of any statistical measure and indicates that every outcome has an equal chance of occurring. Now, let's use this information to solve the problem.
In order to solve this problem, we need to calculate how the probability varies under standard conditions according to the standard deviation. We know that we have a one out of two chance of flipping a coin to heads or tails; therefore, we can write the following probabilities:
We know the standard deviation equals the following:
This means that the probability may vary by this amount; therefore, a fair coin may possess the following probabilities:
Only one coin possesses probabilities outside of this range: "coin 3." This coin posses the following probabilities:
Example Question #3 : Use Probabilities To Make Fair Decisions: Ccss.Math.Content.Hss Md.B.6
Referees at a professional football game need to choose a coin for the pre-game coin toss. Studies have shown that a fair coin possesses the following standard deviation:
They test five coins by flipping them one hundred times and noting how many times each lands on heads or tails. Which of the following coins is least fair according to the standard deviation?
Cannot be determined
Coin 5
Coin 3 and Coin 5
Coin 1 and Coin 2
Coin 1 and Coin 4
Coin 5
This standard relates to our ability to use probability to make fair decisions. Other standards used the expected means formula in order to calculate expected payoffs of games, lottery drawings, insurance policies, business ventures, and others. This information was then used in order to help us make decisions on whether to play games, invest in a company, or choose an insurance policy. Likewise, the essence of this standard is related to fairness or using probabilities to make fair decisions. In order to answer this question we need to discuss probabilities and the concept of fairness in events. First, we will discuss probabilities in a general sense.
A probability is generally defined as the chances or likelihood of an event occurring. It is calculated by identifying two components: the event and the sample space. The event is defined as the favorable outcome or success that we wish to observe. On the other hand, the sample space is defined as the set of all possible outcomes for the event. Mathematically we calculate probabilities by dividing the event by the sample space:
Let's use a simple example: the rolling of a die. We want to know the probability of rolling a one. We know that the sample space is six because there are six sides or outcomes to the die. Also, we know that there is only a single side with a value of one; therefore,
Now, let's convert this into a percentage:
Probabilities expressed in fraction form will have values between zero and one. One indicates that an event will definitely occur, while zero indicates that an event will not occur. Likewise, probabilities expressed as percentages possess values between zero and one hundred percent where probabilities closer to zero are unlikely to occur and those close to one hundred percent are more likely to occur.
According to this logic, we would expect to roll a particular number on a die one out of every six rolls; however, we may roll the same number multiple times or not at all in six rolls. This discrepancy creates a difference between the expected mean and the actual mean. The expected mean is a hypothetical calculation that assumes a very large sample size and no intervening variables (such as differing forces on the roll and changes in the friction of the surface the die is rolled upon between rolls). Under these conditions we can calculate that any number on the die in a "perfect world" should be one out of six. On the other hand the true or actual mean is calculated using "real" date. In these calculations we would roll a die a particular number of times and use it to develop a probability of rolling a particular number. It is important to note that, theoretically, actual means will eventually equal expected means over a large—or near infinite—amount of trials. In other words, over many many rolls we would eventually find that each number on the die has a one in six chance of being rolled.
Now, let's discuss how fairness is described in chance events. In this sense, fairness is associated with randomness. This means that there is no bias being imparted upon the decision in the event. In other words, there is no bias on the part of the individuals nor is there a bias on the part the device being used to produce the outcome of the event. This means that the decision produced from the chance event will be “fair.” It is important to note that deviations from expected probabilities may occur. For instance, a person may roll a six on a fair die six times in a row. According to probability, we would expect a die to land with the six-side facing upwards only once out of six rolls; however, the process of deviation can help explain this phenomenon.
Suppose we rolled a fair die ten times and recorded the distribution. Next, we decided to do this an infinite number of times. We would create a "bell" shaped curve. In this curve the center would represent events that are likely to occur, such as rolling each side of the die on average one out of six times. The tails or extremes of the curve represent unlikely events that may occur but are highly unlikely, such as rolling one side of the die six times in a row. The dead center of the curve represents the mean and the standard deviation measures how far—under standard conditions—we can expect a sample to deviate or vary from the mean. in other words, if a set of dice consistently roll the same value many times, then we can assume that they may have been rigged or altered.
Probability rests upon the concepts of fairness and randomness. These terms are not necessarily synonymous. Fairness relates to the devices being used (e.g. coin, deck of cards, or die). On the other hand, randomness is an essential component of any statistical measure and indicates that every outcome has an equal chance of occurring. Now, let's use this information to solve the problem.
In order to solve this problem, we need to calculate how the probability varies under standard conditions according to the standard deviation. We know that we have a one out of two chance of flipping a coin to heads or tails; therefore, we can write the following probabilities:
We know the standard deviation equals the following:
This means that the probability may vary by this amount; therefore, a fair coin may possess the following probabilities:
The following coins possess probabilities outside of this range:, Coin 1, Coin 2, Coin 3, Coin 4, Coin 5
The coin that is the least fair is: Coin 5
Example Question #2 : Use Probabilities To Make Fair Decisions: Ccss.Math.Content.Hss Md.B.6
Referees at a professional football game need to choose a coin for the pre-game coin toss. Studies have shown that a fair coin possesses the following standard deviation:
They test five coins by flipping them one hundred times and noting how many times each lands on heads or tails. Which of the following coins is least fair according to the standard deviation?
Coin 4
Coin 1
Coin 1 and Coin 2
Cannot be determined
Coin 5
Coin 1
This standard relates to our ability to use probability to make fair decisions. Other standards used the expected means formula in order to calculate expected payoffs of games, lottery drawings, insurance policies, business ventures, and others. This information was then used in order to help us make decisions on whether to play games, invest in a company, or choose an insurance policy. Likewise, the essence of this standard is related to fairness or using probabilities to make fair decisions. In order to answer this question we need to discuss probabilities and the concept of fairness in events. First, we will discuss probabilities in a general sense.
A probability is generally defined as the chances or likelihood of an event occurring. It is calculated by identifying two components: the event and the sample space. The event is defined as the favorable outcome or success that we wish to observe. On the other hand, the sample space is defined as the set of all possible outcomes for the event. Mathematically we calculate probabilities by dividing the event by the sample space:
Let's use a simple example: the rolling of a die. We want to know the probability of rolling a one. We know that the sample space is six because there are six sides or outcomes to the die. Also, we know that there is only a single side with a value of one; therefore,
Now, let's convert this into a percentage:
Probabilities expressed in fraction form will have values between zero and one. One indicates that an event will definitely occur, while zero indicates that an event will not occur. Likewise, probabilities expressed as percentages possess values between zero and one hundred percent where probabilities closer to zero are unlikely to occur and those close to one hundred percent are more likely to occur.
According to this logic, we would expect to roll a particular number on a die one out of every six rolls; however, we may roll the same number multiple times or not at all in six rolls. This discrepancy creates a difference between the expected mean and the actual mean. The expected mean is a hypothetical calculation that assumes a very large sample size and no intervening variables (such as differing forces on the roll and changes in the friction of the surface the die is rolled upon between rolls). Under these conditions we can calculate that any number on the die in a "perfect world" should be one out of six. On the other hand the true or actual mean is calculated using "real" date. In these calculations we would roll a die a particular number of times and use it to develop a probability of rolling a particular number. It is important to note that, theoretically, actual means will eventually equal expected means over a large—or near infinite—amount of trials. In other words, over many many rolls we would eventually find that each number on the die has a one in six chance of being rolled.
Now, let's discuss how fairness is described in chance events. In this sense, fairness is associated with randomness. This means that there is no bias being imparted upon the decision in the event. In other words, there is no bias on the part of the individuals nor is there a bias on the part the device being used to produce the outcome of the event. This means that the decision produced from the chance event will be “fair.” It is important to note that deviations from expected probabilities may occur. For instance, a person may roll a six on a fair die six times in a row. According to probability, we would expect a die to land with the six-side facing upwards only once out of six rolls; however, the process of deviation can help explain this phenomenon.
Suppose we rolled a fair die ten times and recorded the distribution. Next, we decided to do this an infinite number of times. We would create a "bell" shaped curve. In this curve the center would represent events that are likely to occur, such as rolling each side of the die on average one out of six times. The tails or extremes of the curve represent unlikely events that may occur but are highly unlikely, such as rolling one side of the die six times in a row. The dead center of the curve represents the mean and the standard deviation measures how far—under standard conditions—we can expect a sample to deviate or vary from the mean. in other words, if a set of dice consistently roll the same value many times, then we can assume that they may have been rigged or altered.
Probability rests upon the concepts of fairness and randomness. These terms are not necessarily synonymous. Fairness relates to the devices being used (e.g. coin, deck of cards, or die). On the other hand, randomness is an essential component of any statistical measure and indicates that every outcome has an equal chance of occurring. Now, let's use this information to solve the problem.
In order to solve this problem, we need to calculate how the probability varies under standard conditions according to the standard deviation. We know that we have a one out of two chance of flipping a coin to heads or tails; therefore, we can write the following probabilities:
We know the standard deviation equals the following:
This means that the probability may vary by this amount; therefore, a fair coin may possess the following probabilities:
The following coins possess probabilities outside of this range:, Coin 1, Coin 2, Coin 3, Coin 4, Coin 5
The coin that is the least fair is: Coin 1
Example Question #3 : Use Probabilities To Make Fair Decisions: Ccss.Math.Content.Hss Md.B.6
Referees at a professional football game need to choose a coin for the pre-game coin toss. Studies have shown that a fair coin possesses the following standard deviation:
They test five coins by flipping them one hundred times and noting how many times each lands on heads or tails. Which of the following coins is least fair according to the standard deviation?
Coin 2
Coin 1 and Coin 4
Cannot be determined
Coin 3 and Coin 5
Coin 3
Coin 3
This standard relates to our ability to use probability to make fair decisions. Other standards used the expected means formula in order to calculate expected payoffs of games, lottery drawings, insurance policies, business ventures, and others. This information was then used in order to help us make decisions on whether to play games, invest in a company, or choose an insurance policy. Likewise, the essence of this standard is related to fairness or using probabilities to make fair decisions. In order to answer this question we need to discuss probabilities and the concept of fairness in events. First, we will discuss probabilities in a general sense.
A probability is generally defined as the chances or likelihood of an event occurring. It is calculated by identifying two components: the event and the sample space. The event is defined as the favorable outcome or success that we wish to observe. On the other hand, the sample space is defined as the set of all possible outcomes for the event. Mathematically we calculate probabilities by dividing the event by the sample space:
Let's use a simple example: the rolling of a die. We want to know the probability of rolling a one. We know that the sample space is six because there are six sides or outcomes to the die. Also, we know that there is only a single side with a value of one; therefore,
Now, let's convert this into a percentage:
Probabilities expressed in fraction form will have values between zero and one. One indicates that an event will definitely occur, while zero indicates that an event will not occur. Likewise, probabilities expressed as percentages possess values between zero and one hundred percent where probabilities closer to zero are unlikely to occur and those close to one hundred percent are more likely to occur.
According to this logic, we would expect to roll a particular number on a die one out of every six rolls; however, we may roll the same number multiple times or not at all in six rolls. This discrepancy creates a difference between the expected mean and the actual mean. The expected mean is a hypothetical calculation that assumes a very large sample size and no intervening variables (such as differing forces on the roll and changes in the friction of the surface the die is rolled upon between rolls). Under these conditions we can calculate that any number on the die in a "perfect world" should be one out of six. On the other hand the true or actual mean is calculated using "real" date. In these calculations we would roll a die a particular number of times and use it to develop a probability of rolling a particular number. It is important to note that, theoretically, actual means will eventually equal expected means over a large—or near infinite—amount of trials. In other words, over many many rolls we would eventually find that each number on the die has a one in six chance of being rolled.
Now, let's discuss how fairness is described in chance events. In this sense, fairness is associated with randomness. This means that there is no bias being imparted upon the decision in the event. In other words, there is no bias on the part of the individuals nor is there a bias on the part the device being used to produce the outcome of the event. This means that the decision produced from the chance event will be “fair.” It is important to note that deviations from expected probabilities may occur. For instance, a person may roll a six on a fair die six times in a row. According to probability, we would expect a die to land with the six-side facing upwards only once out of six rolls; however, the process of deviation can help explain this phenomenon.
Suppose we rolled a fair die ten times and recorded the distribution. Next, we decided to do this an infinite number of times. We would create a "bell" shaped curve. In this curve the center would represent events that are likely to occur, such as rolling each side of the die on average one out of six times. The tails or extremes of the curve represent unlikely events that may occur but are highly unlikely, such as rolling one side of the die six times in a row. The dead center of the curve represents the mean and the standard deviation measures how far—under standard conditions—we can expect a sample to deviate or vary from the mean. in other words, if a set of dice consistently roll the same value many times, then we can assume that they may have been rigged or altered.
Probability rests upon the concepts of fairness and randomness. These terms are not necessarily synonymous. Fairness relates to the devices being used (e.g. coin, deck of cards, or die). On the other hand, randomness is an essential component of any statistical measure and indicates that every outcome has an equal chance of occurring. Now, let's use this information to solve the problem.
In order to solve this problem, we need to calculate how the probability varies under standard conditions according to the standard deviation. We know that we have a one out of two chance of flipping a coin to heads or tails; therefore, we can write the following probabilities:
We know the standard deviation equals the following:
This means that the probability may vary by this amount; therefore, a fair coin may possess the following probabilities:
The following coins possess probabilities outside of this range:, Coin 1, Coin 3, Coin 5
The coin that is the least fair is: Coin 3
Example Question #4 : Use Probabilities To Make Fair Decisions: Ccss.Math.Content.Hss Md.B.6
Referees at a professional football game need to choose a coin for the pre-game coin toss. Studies have shown that a fair coin possesses the following standard deviation:
s= 0.02
They test five coins by flipping them one hundred times and noting how many times each lands on heads or tails. Which of the following coins is least fair according to the standard deviation?
Coin 1 and Coin 2
Coin 1
Coin 2
Cannot be determined
Coin 4
Coin 4
This standard relates to our ability to use probability to make fair decisions. Other standards used the expected means formula in order to calculate expected payoffs of games, lottery drawings, insurance policies, business ventures, and others. This information was then used in order to help us make decisions on whether to play games, invest in a company, or choose an insurance policy. Likewise, the essence of this standard is related to fairness or using probabilities to make fair decisions. In order to answer this question we need to discuss probabilities and the concept of fairness in events. First, we will discuss probabilities in a general sense.
A probability is generally defined as the chances or likelihood of an event occurring. It is calculated by identifying two components: the event and the sample space. The event is defined as the favorable outcome or success that we wish to observe. On the other hand, the sample space is defined as the set of all possible outcomes for the event. Mathematically we calculate probabilities by dividing the event by the sample space:
Let's use a simple example: the rolling of a die. We want to know the probability of rolling a one. We know that the sample space is six because there are six sides or outcomes to the die. Also, we know that there is only a single side with a value of one; therefore,
Now, let's convert this into a percentage:
Probabilities expressed in fraction form will have values between zero and one. One indicates that an event will definitely occur, while zero indicates that an event will not occur. Likewise, probabilities expressed as percentages possess values between zero and one hundred percent where probabilities closer to zero are unlikely to occur and those close to one hundred percent are more likely to occur.
According to this logic, we would expect to roll a particular number on a die one out of every six rolls; however, we may roll the same number multiple times or not at all in six rolls. This discrepancy creates a difference between the expected mean and the actual mean. The expected mean is a hypothetical calculation that assumes a very large sample size and no intervening variables (such as differing forces on the roll and changes in the friction of the surface the die is rolled upon between rolls). Under these conditions we can calculate that any number on the die in a "perfect world" should be one out of six. On the other hand the true or actual mean is calculated using "real" date. In these calculations we would roll a die a particular number of times and use it to develop a probability of rolling a particular number. It is important to note that, theoretically, actual means will eventually equal expected means over a large—or near infinite—amount of trials. In other words, over many many rolls we would eventually find that each number on the die has a one in six chance of being rolled.
Now, let's discuss how fairness is described in chance events. In this sense, fairness is associated with randomness. This means that there is no bias being imparted upon the decision in the event. In other words, there is no bias on the part of the individuals nor is there a bias on the part the device being used to produce the outcome of the event. This means that the decision produced from the chance event will be “fair.” It is important to note that deviations from expected probabilities may occur. For instance, a person may roll a six on a fair die six times in a row. According to probability, we would expect a die to land with the six-side facing upwards only once out of six rolls; however, the process of deviation can help explain this phenomenon.
Suppose we rolled a fair die ten times and recorded the distribution. Next, we decided to do this an infinite number of times. We would create a "bell" shaped curve. In this curve the center would represent events that are likely to occur, such as rolling each side of the die on average one out of six times. The tails or extremes of the curve represent unlikely events that may occur but are highly unlikely, such as rolling one side of the die six times in a row. The dead center of the curve represents the mean and the standard deviation measures how far—under standard conditions—we can expect a sample to deviate or vary from the mean. in other words, if a set of dice consistently roll the same value many times, then we can assume that they may have been rigged or altered.
Probability rests upon the concepts of fairness and randomness. These terms are not necessarily synonymous. Fairness relates to the devices being used (e.g. coin, deck of cards, or die). On the other hand, randomness is an essential component of any statistical measure and indicates that every outcome has an equal chance of occurring. Now, let's use this information to solve the problem.
In order to solve this problem, we need to calculate how the probability varies under standard conditions according to the standard deviation. We know that we have a one out of two chance of flipping a coin to heads or tails; therefore, we can write the following probabilities:
We know the standard deviation equals the following:
This means that the probability may vary by this amount; therefore, a fair coin may possess the following probabilities:
The following coins possess probabilities outside of this range:, Coin 1, Coin 2, Coin 3, Coin 4, Coin 5
The coin that is the least fair is: Coin 4
Example Question #5 : Use Probabilities To Make Fair Decisions: Ccss.Math.Content.Hss Md.B.6
Referees at a professional football game need to choose a coin for the pre-game coin toss. Studies have shown that a fair coin possesses the following standard deviation:
They test five coins by flipping them one hundred times and noting how many times each lands on heads or tails. Which of the following coins is least fair according to the standard deviation?
Coin 4
Coin 3
Coin 1 and Coin 2
Coin 5
Coin 1 and Coin 4
Coin 4
This standard relates to our ability to use probability to make fair decisions. Other standards used the expected means formula in order to calculate expected payoffs of games, lottery drawings, insurance policies, business ventures, and others. This information was then used in order to help us make decisions on whether to play games, invest in a company, or choose an insurance policy. Likewise, the essence of this standard is related to fairness or using probabilities to make fair decisions. In order to answer this question we need to discuss probabilities and the concept of fairness in events. First, we will discuss probabilities in a general sense.
A probability is generally defined as the chances or likelihood of an event occurring. It is calculated by identifying two components: the event and the sample space. The event is defined as the favorable outcome or success that we wish to observe. On the other hand, the sample space is defined as the set of all possible outcomes for the event. Mathematically we calculate probabilities by dividing the event by the sample space:
Let's use a simple example: the rolling of a die. We want to know the probability of rolling a one. We know that the sample space is six because there are six sides or outcomes to the die. Also, we know that there is only a single side with a value of one; therefore,
Now, let's convert this into a percentage:
Probabilities expressed in fraction form will have values between zero and one. One indicates that an event will definitely occur, while zero indicates that an event will not occur. Likewise, probabilities expressed as percentages possess values between zero and one hundred percent where probabilities closer to zero are unlikely to occur and those close to one hundred percent are more likely to occur.
According to this logic, we would expect to roll a particular number on a die one out of every six rolls; however, we may roll the same number multiple times or not at all in six rolls. This discrepancy creates a difference between the expected mean and the actual mean. The expected mean is a hypothetical calculation that assumes a very large sample size and no intervening variables (such as differing forces on the roll and changes in the friction of the surface the die is rolled upon between rolls). Under these conditions we can calculate that any number on the die in a "perfect world" should be one out of six. On the other hand the true or actual mean is calculated using "real" date. In these calculations we would roll a die a particular number of times and use it to develop a probability of rolling a particular number. It is important to note that, theoretically, actual means will eventually equal expected means over a large—or near infinite—amount of trials. In other words, over many many rolls we would eventually find that each number on the die has a one in six chance of being rolled.
Now, let's discuss how fairness is described in chance events. In this sense, fairness is associated with randomness. This means that there is no bias being imparted upon the decision in the event. In other words, there is no bias on the part of the individuals nor is there a bias on the part the device being used to produce the outcome of the event. This means that the decision produced from the chance event will be “fair.” It is important to note that deviations from expected probabilities may occur. For instance, a person may roll a six on a fair die six times in a row. According to probability, we would expect a die to land with the six-side facing upwards only once out of six rolls; however, the process of deviation can help explain this phenomenon.
Suppose we rolled a fair die ten times and recorded the distribution. Next, we decided to do this an infinite number of times. We would create a "bell" shaped curve. In this curve the center would represent events that are likely to occur, such as rolling each side of the die on average one out of six times. The tails or extremes of the curve represent unlikely events that may occur but are highly unlikely, such as rolling one side of the die six times in a row. The dead center of the curve represents the mean and the standard deviation measures how far—under standard conditions—we can expect a sample to deviate or vary from the mean. in other words, if a set of dice consistently roll the same value many times, then we can assume that they may have been rigged or altered.
Probability rests upon the concepts of fairness and randomness. These terms are not necessarily synonymous. Fairness relates to the devices being used (e.g. coin, deck of cards, or die). On the other hand, randomness is an essential component of any statistical measure and indicates that every outcome has an equal chance of occurring. Now, let's use this information to solve the problem.
In order to solve this problem, we need to calculate how the probability varies under standard conditions according to the standard deviation. We know that we have a one out of two chance of flipping a coin to heads or tails; therefore, we can write the following probabilities:
We know the standard deviation equals the following:
This means that the probability may vary by this amount; therefore, a fair coin may possess the following probabilities:
The following coins possess probabilities outside of this range:, Coin 1, Coin 2, Coin 3, Coin 4, Coin 5
The coin that is the least fair is: Coin 4
Example Question #6 : Use Probabilities To Make Fair Decisions: Ccss.Math.Content.Hss Md.B.6
Referees at a professional football game need to choose a coin for the pre-game coin toss. Studies have shown that a fair coin possesses the following standard deviation:
They test five coins by flipping them one hundred times and noting how many times each lands on heads or tails. Which of the following coins is least fair according to the standard deviation?
Coin 5
Coin 1
Coin 3
Coin 2
Coin 4
Coin 4
This standard relates to our ability to use probability to make fair decisions. Other standards used the expected means formula in order to calculate expected payoffs of games, lottery drawings, insurance policies, business ventures, and others. This information was then used in order to help us make decisions on whether to play games, invest in a company, or choose an insurance policy. Likewise, the essence of this standard is related to fairness or using probabilities to make fair decisions. In order to answer this question we need to discuss probabilities and the concept of fairness in events. First, we will discuss probabilities in a general sense.
A probability is generally defined as the chances or likelihood of an event occurring. It is calculated by identifying two components: the event and the sample space. The event is defined as the favorable outcome or success that we wish to observe. On the other hand, the sample space is defined as the set of all possible outcomes for the event. Mathematically we calculate probabilities by dividing the event by the sample space:
Let's use a simple example: the rolling of a die. We want to know the probability of rolling a one. We know that the sample space is six because there are six sides or outcomes to the die. Also, we know that there is only a single side with a value of one; therefore,
Now, let's convert this into a percentage:
Probabilities expressed in fraction form will have values between zero and one. One indicates that an event will definitely occur, while zero indicates that an event will not occur. Likewise, probabilities expressed as percentages possess values between zero and one hundred percent where probabilities closer to zero are unlikely to occur and those close to one hundred percent are more likely to occur.
According to this logic, we would expect to roll a particular number on a die one out of every six rolls; however, we may roll the same number multiple times or not at all in six rolls. This discrepancy creates a difference between the expected mean and the actual mean. The expected mean is a hypothetical calculation that assumes a very large sample size and no intervening variables (such as differing forces on the roll and changes in the friction of the surface the die is rolled upon between rolls). Under these conditions we can calculate that any number on the die in a "perfect world" should be one out of six. On the other hand the true or actual mean is calculated using "real" date. In these calculations we would roll a die a particular number of times and use it to develop a probability of rolling a particular number. It is important to note that, theoretically, actual means will eventually equal expected means over a large—or near infinite—amount of trials. In other words, over many many rolls we would eventually find that each number on the die has a one in six chance of being rolled.
Now, let's discuss how fairness is described in chance events. In this sense, fairness is associated with randomness. This means that there is no bias being imparted upon the decision in the event. In other words, there is no bias on the part of the individuals nor is there a bias on the part the device being used to produce the outcome of the event. This means that the decision produced from the chance event will be “fair.” It is important to note that deviations from expected probabilities may occur. For instance, a person may roll a six on a fair die six times in a row. According to probability, we would expect a die to land with the six-side facing upwards only once out of six rolls; however, the process of deviation can help explain this phenomenon.
Suppose we rolled a fair die ten times and recorded the distribution. Next, we decided to do this an infinite number of times. We would create a "bell" shaped curve. In this curve the center would represent events that are likely to occur, such as rolling each side of the die on average one out of six times. The tails or extremes of the curve represent unlikely events that may occur but are highly unlikely, such as rolling one side of the die six times in a row. The dead center of the curve represents the mean and the standard deviation measures how far—under standard conditions—we can expect a sample to deviate or vary from the mean. in other words, if a set of dice consistently roll the same value many times, then we can assume that they may have been rigged or altered.
Probability rests upon the concepts of fairness and randomness. These terms are not necessarily synonymous. Fairness relates to the devices being used (e.g. coin, deck of cards, or die). On the other hand, randomness is an essential component of any statistical measure and indicates that every outcome has an equal chance of occurring. Now, let's use this information to solve the problem.
In order to solve this problem, we need to calculate how the probability varies under standard conditions according to the standard deviation. We know that we have a one out of two chance of flipping a coin to heads or tails; therefore, we can write the following probabilities:
We know the standard deviation equals the following:
This means that the probability may vary by this amount; therefore, a fair coin may possess the following probabilities:
The following coins possess probabilities outside of this range:, Coin 2, Coin 4, Coin 5
The coin that is the least fair is: Coin 4
Example Question #3 : Use Probabilities To Make Fair Decisions: Ccss.Math.Content.Hss Md.B.6
Referees at a professional football game need to choose a coin for the pre-game coin toss. Studies have shown that a fair coin possesses the following standard deviation:
They test five coins by flipping them one hundred times and noting how many times each lands on heads or tails. Which of the following coins is least fair according to the standard deviation?
Coin 5 and Coin 2
Coin 1
Coin 5
Coin 4
Coin 3 and Coin 4
Coin 1
This standard relates to our ability to use probability to make fair decisions. Other standards used the expected means formula in order to calculate expected payoffs of games, lottery drawings, insurance policies, business ventures, and others. This information was then used in order to help us make decisions on whether to play games, invest in a company, or choose an insurance policy. Likewise, the essence of this standard is related to fairness or using probabilities to make fair decisions. In order to answer this question we need to discuss probabilities and the concept of fairness in events. First, we will discuss probabilities in a general sense.
A probability is generally defined as the chances or likelihood of an event occurring. It is calculated by identifying two components: the event and the sample space. The event is defined as the favorable outcome or success that we wish to observe. On the other hand, the sample space is defined as the set of all possible outcomes for the event. Mathematically we calculate probabilities by dividing the event by the sample space:
Let's use a simple example: the rolling of a die. We want to know the probability of rolling a one. We know that the sample space is six because there are six sides or outcomes to the die. Also, we know that there is only a single side with a value of one; therefore,
Now, let's convert this into a percentage:
Probabilities expressed in fraction form will have values between zero and one. One indicates that an event will definitely occur, while zero indicates that an event will not occur. Likewise, probabilities expressed as percentages possess values between zero and one hundred percent where probabilities closer to zero are unlikely to occur and those close to one hundred percent are more likely to occur.
According to this logic, we would expect to roll a particular number on a die one out of every six rolls; however, we may roll the same number multiple times or not at all in six rolls. This discrepancy creates a difference between the expected mean and the actual mean. The expected mean is a hypothetical calculation that assumes a very large sample size and no intervening variables (such as differing forces on the roll and changes in the friction of the surface the die is rolled upon between rolls). Under these conditions we can calculate that any number on the die in a "perfect world" should be one out of six. On the other hand the true or actual mean is calculated using "real" date. In these calculations we would roll a die a particular number of times and use it to develop a probability of rolling a particular number. It is important to note that, theoretically, actual means will eventually equal expected means over a large—or near infinite—amount of trials. In other words, over many many rolls we would eventually find that each number on the die has a one in six chance of being rolled.
Now, let's discuss how fairness is described in chance events. In this sense, fairness is associated with randomness. This means that there is no bias being imparted upon the decision in the event. In other words, there is no bias on the part of the individuals nor is there a bias on the part the device being used to produce the outcome of the event. This means that the decision produced from the chance event will be “fair.” It is important to note that deviations from expected probabilities may occur. For instance, a person may roll a six on a fair die six times in a row. According to probability, we would expect a die to land with the six-side facing upwards only once out of six rolls; however, the process of deviation can help explain this phenomenon.
Suppose we rolled a fair die ten times and recorded the distribution. Next, we decided to do this an infinite number of times. We would create a "bell" shaped curve. In this curve the center would represent events that are likely to occur, such as rolling each side of the die on average one out of six times. The tails or extremes of the curve represent unlikely events that may occur but are highly unlikely, such as rolling one side of the die six times in a row. The dead center of the curve represents the mean and the standard deviation measures how far—under standard conditions—we can expect a sample to deviate or vary from the mean. in other words, if a set of dice consistently roll the same value many times, then we can assume that they may have been rigged or altered.
Probability rests upon the concepts of fairness and randomness. These terms are not necessarily synonymous. Fairness relates to the devices being used (e.g. coin, deck of cards, or die). On the other hand, randomness is an essential component of any statistical measure and indicates that every outcome has an equal chance of occurring. Now, let's use this information to solve the problem.
In order to solve this problem, we need to calculate how the probability varies under standard conditions according to the standard deviation. We know that we have a one out of two chance of flipping a coin to heads or tails; therefore, we can write the following probabilities:
We know the standard deviation equals the following:
This means that the probability may vary by this amount; therefore, a fair coin may possess the following probabilities:
The following coins possess probabilities outside of this range:, Coin 1, Coin 2, Coin 4, Coin 5
The coin that is the least fair is: Coin 1
All Common Core: High School - Statistics and Probability Resources
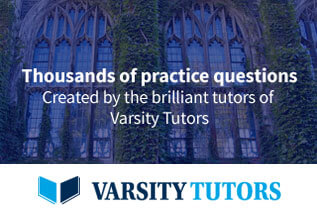